Open Access
ARTICLE
Prediction of Time Series Empowered with a Novel SREKRLS Algorithm
1 School of Computer Science, Minhaj University Lahore, Lahore, 54000, Pakistan
2 Prince Sultan University, Riyadh, 11586, Saudi Arabia
3 Department of Computer Science, Riphah International University Lahore Campus, Lahore, 54000, Pakistan
4 Department of Basic Sciences, Deanship of Common First Year, Jouf University, Sakaka, Aljouf, 72341, Saudi Arabia
5 Directorate of IT, The Islamia University of Bahawalpur, Bahawalpur, 63100, Pakistan
6 Department of Computer Science, University of Management and Technology, Lahore, 54770, Pakistan
7 PUCIT, University of the Punjab, Lahore, 54000, Pakistan
* Corresponding Author: Muhammad Adnan Khan. Email:
Computers, Materials & Continua 2021, 67(2), 1413-1427. https://doi.org/10.32604/cmc.2021.015099
Received 06 November 2020; Accepted 03 December 2020; Issue published 05 February 2021
Abstract
For the unforced dynamical non-linear statespace model, a new Q1 and efficient square root extended kernel recursive least square estimation algorithm is developed in this article. The proposed algorithm lends itself towards the parallel implementation as in the FPGA systems. With the help of an ortho-normal triangularization method, which relies on numerically stable givens rotation, matrix inversion causes a computational burden, is reduced. Matrix computation possesses many excellent numerical properties such as singularity, symmetry, skew symmetry, and triangularity is achieved by using this algorithm. The proposed method is validated for the prediction of stationary and non-stationary MackeyGlass Time Series, along with that a component in the x-direction of the Lorenz Times Series is also predicted to illustrate its usefulness. By the learning curves regarding mean square error (MSE) are witnessed for demonstration with prediction performance of the proposed algorithm from where it’s concluded that the proposed algorithm performs better than EKRLS. This new SREKRLS based design positively offers an innovative era towards non-linear systolic arrays, which is efficient in developing very-large-scale integration (VLSI) applications with non-linear input data. Multiple experiments are carried out to validate the reliability, effectiveness, and applicability of the proposed algorithm and with different noise levels compared to the Extended kernel recursive least-squares (EKRLS) algorithm.Keywords
Cite This Article
Citations
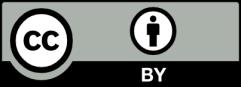