Open Access
ARTICLE
Industrial Food Quality Analysis Using New k-Nearest-Neighbour methods
1 Laboratory of Statistics and Stochastic Processes, University of Djillali Liabes, Sidi Bel Abbes, 22000, Algeria.
2 Department of Mathematics, College of Science, King Khalid University, Abha, 62529, Saudi Arabia
3 Statistical Research and Studies Support Unit, King Khalid University, Abha, 62529, Saudi Arabia
4 Faculté des sciences de Tunis, Laboratoire des Réseaux Intelligents et Nanotechnologie, Tunis, Tunisia
* Corresponding Author: Mohammed Kadi Attouch. Email:
Computers, Materials & Continua 2021, 67(2), 2681-2694. https://doi.org/10.32604/cmc.2021.015469
Received 23 November 2020; Accepted 01 January 2021; Issue published 05 February 2021
Abstract
The problem of predicting continuous scalar outcomes from functional predictors has received high levels of interest in recent years in many fields, especially in the food industry. The k-nearest neighbor (k-NN) method of Near-Infrared Reflectance (NIR) analysis is practical, relatively easy to implement, and becoming one of the most popular methods for conducting food quality based on NIR data. The k-NN is often named k nearest neighbor classifier when it is used for classifying categorical variables, while it is called k-nearest neighbor regression when it is applied for predicting noncategorical variables. The objective of this paper is to use the functional Near-Infrared Reflectance (NIR) spectroscopy approach to predict some chemical components with some modern statistical models based on the kernel and k-Nearest Neighbour procedures. In this paper, three NIR spectroscopy datasets are used as examples, namely Cookie dough, sugar, and tecator data. Specifically, we propose three models for this kind of data which are Functional Nonparametric Regression, Functional Robust Regression, and Functional Relative Error Regression, with both kernel and k-NN approaches to compare between them. The experimental result shows the higher efficiency of k-NN predictor over the kernel predictor. The predictive power of the k-NN method was compared with that of the kernel method, and several real data sets were used to determine the predictive power of both methods.Keywords
Cite This Article
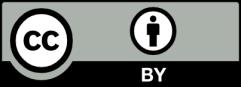