Open Access
ARTICLE
Multi-Span and Multiple Relevant Time Series Prediction Based on Neighborhood Rough Set
1 School of Computer Science and Technology, Nanjing TECH University, Nanjing, 211816, China
2 School of Computer Science and Engineering, Kyungpook National University, Daegu, 41566, Korea
* Corresponding Author: Zixu An. Email:
Computers, Materials & Continua 2021, 67(3), 3765-3780. https://doi.org/10.32604/cmc.2021.012422
Received 30 August 2020; Accepted 31 December 2020; Issue published 01 March 2021
Abstract
Rough set theory has been widely researched for time series prediction problems such as rainfall runoff. Accurate forecasting of rainfall runoff is a long standing but still mostly significant problem for water resource planning and management, reservoir and river regulation. Most research is focused on constructing the better model for improving prediction accuracy. In this paper, a rainfall runoff forecast model based on the variable-precision fuzzy neighborhood rough set (VPFNRS) is constructed to predict Watershed runoff value. Fuzzy neighborhood rough set define the fuzzy decision of a sample by using the concept of fuzzy neighborhood. The fuzzy neighborhood rough set model with variable-precision can reduce the redundant attributes, and the essential equivalent data can improve the predictive capabilities of model. Meanwhile VFPFNRS can handle the numerical data, while it also deals well with the noise data. In the discussed approach, VPFNRS is used to reduce superfluous attributes of the original data, the compact data are employed for predicting the rainfall runoff. The proposed method is examined utilizing data in the Luo River Basin located in Guangdong, China. The prediction accuracy is compared with that of support vector machines and long short-term memory (LSTM). The experiments show that the method put forward achieves a higher predictive performance.Keywords
Cite This Article
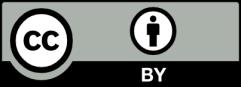