Open Access
ARTICLE
Paddy Leaf Disease Detection Using an Optimized Deep Neural Network
1 Department of Computer Science & Engineering, University College of Engineering, BIT Campus, Anna University, Tiruchirappalli, 620024, India
2 School of Computing, SRM Institute of Science and Technology, Kattankulathur, 603203, India
3 Department of Electronics and Communication Engineering, University College of Engineering, BIT Campus, Anna University, Tiruchirappalli, 620024, India
4 Department of Electronics & Communication Engineering, SSM Institute of Engineering and Technology, Dindigul, 624002, India
5 Department of Mechanical Engineering, Rohini College of Engineering and Technology, Palkulam, 629401, India
6 Department of ECE, Karpagam Academy of Higher Education, Coimbatore, 641021, India
7 Department of Electrical and Electronics Engineering, SRM Institute of Science and Technology, Chennai, 603203, India
* Corresponding Author: Shankarnarayanan Nalini. Email:
Computers, Materials & Continua 2021, 68(1), 1117-1128. https://doi.org/10.32604/cmc.2021.012431
Received 30 August 2020; Accepted 04 February 2021; Issue published 22 March 2021
Abstract
Precision Agriculture is a concept of farm management which makes use of IoT and networking concepts to improve the crop. Plant diseases are one of the underlying causes in the decrease in the number of quantity and quality of the farming crops. Recognition of diseases from the plant images is an active research topic which makes use of machine learning (ML) approaches. A novel deep neural network (DNN) classification model is proposed for the identification of paddy leaf disease using plant image data. Classification errors were minimized by optimizing weights and biases in the DNN model using a crow search algorithm (CSA) during both the standard pre-training and fine-tuning processes. This DNN-CSA architecture enables the use of simplistic statistical learning techniques with a decreased computational workload, ensuring high classification accuracy. Paddy leaf images were first preprocessed, and the areas indicative of disease were initially extracted using a k-means clustering method. Thresholding was then applied to eliminate regions not indicative of disease. Next, a set of features were extracted from the previously isolated diseased regions. Finally, the classification accuracy and efficiency of the proposed DNN-CSA model were verified experimentally and shown to be superior to a support vector machine with multiple cross-fold validations.Keywords
Cite This Article
Citations
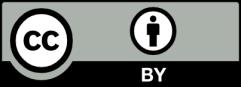