Open Access
ARTICLE
Multi Sensor-Based Implicit User Identification
1 Department of Computer Science, National University of Computer and Emerging Sciences, Chiniot, 35400, Pakistan
2 Department of Computer Science, Bahauddin Zakariya University, Multan, 66000, Pakistan
3 Institute of Software Development and Engineering, Innopolis University, Innopolis, 420500, Russia
4 Dipartimento di Matematica e Informatica—MIFT, University of Messina, Messina, 98121, Italy
5 Department of Computing and Mathematics, Manchester Metropolitan University, Manchester, UK
* Corresponding Author: Muhammad Ahmad. Email:
(This article belongs to the Special Issue: Emerging Applications of Artificial Intelligence, Machine learning and Data Science)
Computers, Materials & Continua 2021, 68(2), 1673-1692. https://doi.org/10.32604/cmc.2021.016232
Received 28 December 2020; Accepted 06 February 2021; Issue published 13 April 2021
Abstract
Smartphones have ubiquitously integrated into our home and work environments, however, users normally rely on explicit but inefficient identification processes in a controlled environment. Therefore, when a device is stolen, a thief can have access to the owner’s personal information and services against the stored passwords. As a result of this potential scenario, this work proposes an automatic legitimate user identification system based on gait biometrics extracted from user walking patterns captured by smartphone sensors. A set of preprocessing schemes are applied to calibrate noisy and invalid samples and augment the gait-induced time and frequency domain features, then further optimized using a non-linear unsupervised feature selection method. The selected features create an underlying gait biometric representation able to discriminate among individuals and identify them uniquely. Different classifiers are adopted to achieve accurate legitimate user identification. Extensive experiments on a group of 16 individuals in an indoor environment show the effectiveness of the proposed solution: with 5 to 70 samples per window, KNN and bagging classifiers achieve 87–99% accuracy, 82–98% for ELM, and 81–94% for SVM. The proposed pipeline achieves a 100% true positive and 0% false-negative rate for almost all classifiers.Keywords
Cite This Article
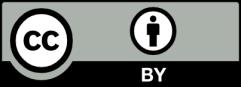