Open Access
ARTICLE
Data and Machine Learning Fusion Architecture for Cardiovascular Disease Prediction
1 School of Computer Science, National College of Business Administration & Economics, Lahore, 54000, Pakistan
2 College of Computer and Information Sciences, Jouf University, Sakaka, 72341, Saudi Arabia
3 Department of Computer Science, Virtual University of Pakistan, Lahore, 54000, Pakistan
4 Riphah School of Computing & Innovation, Riphah International University, Lahore Campus, Lahore, 54000, Pakistan
5 Department of Computer Science, Lahore Garrison University, Lahore, 54000, Pakistan
6 Department of Computer Science, Minhaj University Lahore, Lahore, 54000, Pakistan
7 College of Engineering, Al Ain University, Abu Dhabi, 112612, UAE
8 Department of Computer Science, Faculty of Computers and Artificial Intelligence, Cairo University, 12613, Egypt
* Corresponding Author: Muhammad Adnan Khan. Email:
Computers, Materials & Continua 2021, 69(2), 2717-2731. https://doi.org/10.32604/cmc.2021.019013
Received 28 March 2021; Accepted 29 April 2021; Issue published 21 July 2021
Abstract
Heart disease, which is also known as cardiovascular disease, includes various conditions that affect the heart and has been considered a major cause of death over the past decades. Accurate and timely detection of heart disease is the single key factor for appropriate investigation, treatment, and prescription of medication. Emerging technologies such as fog, cloud, and mobile computing provide substantial support for the diagnosis and prediction of fatal diseases such as diabetes, cancer, and cardiovascular disease. Cloud computing provides a cost-efficient infrastructure for data processing, storage, and retrieval, with much of the extant research recommending machine learning (ML) algorithms for generating models for sample data. ML is considered best suited to explore hidden patterns, which is ultimately helpful for analysis and prediction. Accordingly, this study combines cloud computing with ML, collecting datasets from different geographical areas and applying fusion techniques to maintain data accuracy and consistency for the ML algorithms. Our recommended model considered three ML techniques: Artificial Neural Network, Decision Tree, and Naïve Bayes. Real-time patient data were extracted using the fuzzy-based model stored in the cloud.Keywords
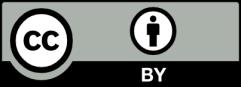