Open Access
ARTICLE
Gastrointestinal Tract Infections Classification Using Deep Learning
1 Department of Computer Science, COMSATS University Islamabad, Wah Campus, 47040, Pakistan
2 Department of Computer Science, HITEC University Taxila, Taxila, 47080, Pakistan
3 Department of Computer Science and Engineering, Soonchunhyang University, Asan, Korea
* Corresponding Author: Yunyoung Nam. Email:
(This article belongs to the Special Issue: Artificial Intelligence and IoT based intelligent systems using high performance computing for Medical applications.)
Computers, Materials & Continua 2021, 69(3), 3239-3257. https://doi.org/10.32604/cmc.2021.015920
Received 14 December 2020; Accepted 29 March 2021; Issue published 24 August 2021
Abstract
Automatic gastrointestinal (GI) tract disease recognition is an important application of biomedical image processing. Conventionally, microscopic analysis of pathological tissue is used to detect abnormal areas of the GI tract. The procedure is subjective and results in significant inter-/intra-observer variations in disease detection. Moreover, a huge frame rate in video endoscopy is an overhead for the pathological findings of gastroenterologists to observe every frame with a detailed examination. Consequently, there is a huge demand for a reliable computer-aided diagnostic system (CADx) for diagnosing GI tract diseases. In this work, a CADx was proposed for the diagnosis and classification of GI tract diseases. A novel framework is presented where preprocessing (LAB color space) is performed first; then local binary patterns (LBP) or texture and deep learning (inceptionNet, ResNet50, and VGG-16) features are fused serially to improve the prediction of the abnormalities in the GI tract. Additionally, principal component analysis (PCA), entropy, and minimum redundancy and maximum relevance (mRMR) feature selection methods were analyzed to acquire the optimized characteristics, and various classifiers were trained using the fused features. Open-source color image datasets (KVASIR, NERTHUS, and stomach ULCER) were used for performance evaluation. The study revealed that the subspace discriminant classifier provided an efficient result with 95.02% accuracy on the KVASIR dataset, which proved to be better than the existing state-of-the-art approaches.Keywords
Cite This Article
Citations
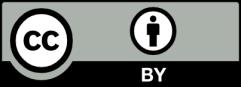