Open Access
ARTICLE
An Intelligent Gestational Diabetes Diagnosis Model Using Deep Stacked Autoencoder
1 SRC, SASTRA Deemed to be University, Kumbakonam, Tamil Nadu, 612001, India
2 Consultant Obstetrician and Gynecologist, Anbu Hospital and Nalam Clinic, Kumbakonam, Tamil Nadu, 612001, India
* Corresponding Author: A. Sumathi. Email:
Computers, Materials & Continua 2021, 69(3), 3109-3126. https://doi.org/10.32604/cmc.2021.017612
Received 04 February 2021; Accepted 29 March 2021; Issue published 24 August 2021
Abstract
Gestational Diabetes Mellitus (GDM) is one of the commonly occurring diseases among women during pregnancy. Oral Glucose Tolerance Test (OGTT) is followed universally in the diagnosis of GDM diagnosis at early pregnancy which is costly and ineffective. So, there is a need to design an effective and automated GDM diagnosis and classification model. The recent developments in the field of Deep Learning (DL) are useful in diagnosing different diseases. In this view, the current research article presents a new outlier detection with deep-stacked Autoencoder (OD-DSAE) model for GDM diagnosis and classification. The goal of the proposed OD-DSAE model is to find out those mothers with high risks and make them undergo earlier diagnosis, monitoring, and treatment compared to low-risk women. The presented OD-DSAE model involves three major processes namely, preprocessing, outlier detection, and classification. In the first step i.e., data preprocessing, there exists three stages namely, format conversion, class labelling, and missing value replacement using k-nearest neighbors (KNN) model. Outliers are superior values which considerably varies from other data observations. So, it might represent the variability in measurement, experimental errors or novelty too. So, Hierarchical Clustering (HC)-based outlier detection technique is incorporated in OD-DSAE model, and thereby classification performance can be improved. The proposed model was simulated using Python 3.6.5 on a dataset collected by the researcher themselves. A series of experiments was conducted and the results were investigated under different aspects. The experimental outcomes inferred that the OD-DSAE model has outperformed the compared methods and achieved high precision of 96.17%, recall of 98.69%, specificity of 89.50%, accuracy of 96.18%, and F-score of 97.41%.Keywords
Cite This Article
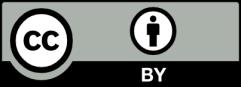