Open Access
ARTICLE
FPGA Implementation of Deep Leaning Model for Video Analytics
Mahendra College of Engineering, Salem, India
* Corresponding Author: P. N. Palanisamy. Email:
Computers, Materials & Continua 2022, 71(1), 791-808. https://doi.org/10.32604/cmc.2022.019921
Received 01 May 2021; Accepted 12 July 2021; Issue published 03 November 2021
Abstract
In recent years, deep neural networks have become a fascinating and influential research subject, and they play a critical role in video processing and analytics. Since, video analytics are predominantly hardware centric, exploration of implementing the deep neural networks in the hardware needs its brighter light of research. However, the computational complexity and resource constraints of deep neural networks are increasing exponentially by time. Convolutional neural networks are one of the most popular deep learning architecture especially for image classification and video analytics. But these algorithms need an efficient implement strategy for incorporating more real time computations in terms of handling the videos in the hardware. Field programmable Gate arrays (FPGA) is thought to be more advantageous in implementing the convolutional neural networks when compared to Graphics Processing Unit (GPU) in terms of energy efficient and low computational complexity. But still, an intelligent architecture is required for implementing the CNN in FPGA for processing the videos. This paper introduces a modern high-performance, energy-efficient Bat Pruned Ensembled Convolutional networks (BPEC-CNN) for processing the video in the hardware. The system integrates the Bat Evolutionary Pruned layers for CNN and implements the new shared Distributed Filtering Structures (DFS) for handing the filter layers in CNN with pipelined data-path in FPGA. In addition, the proposed system adopts the hardware-software co-design methodology for an energy efficiency and less computational complexity. The extensive experimentations are carried out using CASIA video datasets with ARTIX-7 FPGA boards (number) and various algorithms centric parameters such as accuracy, sensitivity, specificity and architecture centric parameters such as the power, area and throughput are analyzed. These results are then compared with the existing pruned CNN architectures such as CNN-Prunner in which the proposed architecture has been shown 25% better performance than the existing architectures.Keywords
Cite This Article
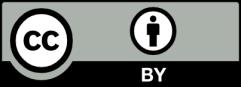