Open Access
ARTICLE
Deep Deterministic Policy Gradient to Regulate Feedback Control Systems Using Reinforcement Learning
1 Department of Electrical & Computer Engineering, COMSATS University Islamabad, Lahore Campus, 54000, Pakistan
2 Department of Electrical Engineering, Government College University, Lahore, 54000, Pakistan
3 Industrial Engineering Department, College of Engineering, King Saud University, P.O. Box 800, Riyadh, 11421, Saudi Arabia
4 Department of Information and Communication Engineering, Yeungnam University, Gyeongsan, 38541, Korea
* Corresponding Author: Muhammad Shafiq. Email:
Computers, Materials & Continua 2022, 71(1), 1153-1169. https://doi.org/10.32604/cmc.2022.021917
Received 19 July 2021; Accepted 07 September 2021; Issue published 03 November 2021
Abstract
Controlling feedback control systems in continuous action spaces has always been a challenging problem. Nevertheless, reinforcement learning is mainly an area of artificial intelligence (AI) because it has been used in process control for more than a decade. However, the existing algorithms are unable to provide satisfactory results. Therefore, this research uses a reinforcement learning (RL) algorithm to manage the control system. We propose an adaptive speed control of the motor system based on depth deterministic strategy gradient (DDPG). The actor-critic scenario using DDPG is implemented to build the RL agent. In addition, a framework has been created for traditional feedback control systems to make RL implementation easier for control systems. The RL algorithms are robust and proficient in using trial and error to search for the best strategy. Our proposed algorithm is a deep deterministic policy gradient, in which a large amount of training data trains the agent. Once the system is trained, the agent can automatically adjust the control parameters. The algorithm has been developed using Python 3.6 and the simulation results are evaluated in the MATLAB/Simulink environment. The performance of the proposed RL algorithm is compared with a proportional integral derivative (PID) controller and a linear quadratic regulator (LQR) controller. The simulation results of the proposed scheme are promising for the feedback control problems.Keywords
Cite This Article
J. Arshad, A. Khan, M. Aftab, M. Hussain, A. Ur Rehman et al., "Deep deterministic policy gradient to regulate feedback control systems using reinforcement learning," Computers, Materials & Continua, vol. 71, no.1, pp. 1153–1169, 2022. https://doi.org/10.32604/cmc.2022.021917Citations
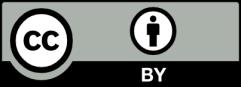