Open Access
ARTICLE
Research on Optimization of Random Forest Algorithm Based on Spark
1 Hebei University of Economics and Business, Shijiazhuang, 050061, China
2 Griffith University, Brisbane, 4222, Australia
* Corresponding Author: Zhanfeng Zhang. Email:
Computers, Materials & Continua 2022, 71(2), 3721-3731. https://doi.org/10.32604/cmc.2022.015378
Received 18 November 2020; Accepted 04 March 2021; Issue published 07 December 2021
Abstract
As society has developed, increasing amounts of data have been generated by various industries. The random forest algorithm, as a classification algorithm, is widely used because of its superior performance. However, the random forest algorithm uses a simple random sampling feature selection method when generating feature subspaces which cannot distinguish redundant features, thereby affecting its classification accuracy, and resulting in a low data calculation efficiency in the stand-alone mode. In response to the aforementioned problems, related optimization research was conducted with Spark in the present paper. This improved random forest algorithm performs feature extraction according to the calculated feature importance to form a feature subspace. When generating a random forest model, it selects decision trees based on the similarity and classification accuracy of different decision. Experimental results reveal that compared with the original random forest algorithm, the improved algorithm proposed in the present paper exhibited a higher classification accuracy rate and could effectively classify data.Keywords
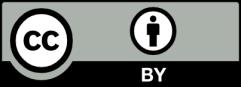