Open Access
ARTICLE
Optimal Hybrid Feature Extraction with Deep Learning for COVID-19 Classifications
1 Department of Information Systems, College of Science & Art at Mahayil, King Khalid University, Saudi Arabia
2 Department of Information Systems, Prince Sultan University, Riyadh, 11586, Saudi Arabia
3 Department of Computer Science, King Khalid University, Muhayel Aseer, 62529, Saudi Arabia
4 Faculty of Computer and IT, Sana'a University, Sana'a, 61101, Yemen
5 Department of Computer and Self Development, Preparatory Year Deanship, Prince Sattam bin Abdulaziz University, AlKharj, Saudi Arabia
6 Faculty of Arts and Science, Najran University, Sharourah, Saudi Arabia
7 Department of Natural and Applied Sciences, College of Community-Aflaj, Prince Sattam bin Abdulaziz University, Saudi Arabia
* Corresponding Author: Manar Ahmed Hamza. Email:
Computers, Materials & Continua 2022, 71(3), 6257-6273. https://doi.org/10.32604/cmc.2022.024312
Received 13 October 2021; Accepted 16 December 2021; Issue published 14 January 2022
Abstract
Novel coronavirus 2019 (COVID-19) has affected the people's health, their lifestyle and economical status across the globe. The application of advanced Artificial Intelligence (AI) methods in combination with radiological imaging is useful in accurate detection of the disease. It also assists the physicians to take care of remote villages too. The current research paper proposes a novel automated COVID-19 analysis method with the help of Optimal Hybrid Feature Extraction (OHFE) and Optimal Deep Neural Network (ODNN) called OHFE-ODNN from chest x-ray images. The objective of the presented technique is for performing binary and multi-class classification of COVID-19 analysis from chest X-ray image. The presented OHFE-ODNN method includes a sequence of procedures such as Median Filtering (MF)-based pre-processed, feature extraction and finally, binary (COVID/Non-COVID) and multiclass (Normal, COVID, SARS) classification. Besides, in OHFE-based feature extraction, Gray Level Co-occurrence Matrix (GLCM) and Histogram of Gradients (HOG) are integrated together. The presented OHFE-ODNN model includes Squirrel Search Algorithm (SSA) for fine-tuning the parameters of DNN. The performance of the presented OHFE-ODNN technique is conducted using chest x-rays dataset. The presented OHFE-ODNN method classified the binary classes effectively with a maximum precision of 95.82%, accuracy of 94.01% and F-score of 96.61%. Besides, multiple classes were classified proficiently by OHFE-ODNN model with a precision of 95.63%, accuracy of 95.60% and an F-score of 95.73%.Keywords
Cite This Article
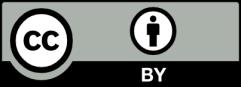