Open Access
ARTICLE
An Adaptive Classifier Based Approach for Crowd Anomaly Detection
School of Computer Science and Engineering, Vellore Institute of Technology, Chennai, 600 127, India
* Corresponding Author: P. S. Nithya Darisini. Email:
(This article belongs to the Special Issue: Innovative Technology For Machine Intelligence)
Computers, Materials & Continua 2022, 72(1), 349-364. https://doi.org/10.32604/cmc.2022.023935
Received 27 September 2021; Accepted 20 December 2021; Issue published 24 February 2022
Abstract
Crowd Anomaly Detection has become a challenge in intelligent video surveillance system and security. Intelligent video surveillance systems make extensive use of data mining, machine learning and deep learning methods. In this paper a novel approach is proposed to identify abnormal occurrences in crowded situations using deep learning. In this approach, Adaptive GoogleNet Neural Network Classifier with Multi-Objective Whale Optimization Algorithm are applied to predict the abnormal video frames in the crowded scenes. We use multiple instance learning (MIL) to dynamically develop a deep anomalous ranking framework. This technique predicts higher anomalous values for abnormal video frames by treating regular and irregular video bags and video sections. We use the multi-objective whale optimization algorithm to optimize the entire process and get the best results. The performance parameters such as accuracy, precision, recall, and F-score are considered to evaluate the proposed technique using the Python simulation tool. Our simulation results show that the proposed method performs better than the conventional methods on the public live video dataset.Keywords
Cite This Article
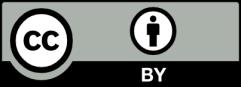