Open Access
ARTICLE
Efficient Computation Offloading of IoT-Based Workflows Using Discrete Teaching Learning-Based Optimization
1 Department of Computer Science, Faculty of Computers and Informatics, Suez Canal University, Ismailia, Egypt
2 Department of Information Technology, College of Computer Science at AlKamil, University of Jeddah, Jeddah, Saudi Arabia
* Corresponding Author: Mohamed K. Hussein. Email:
Computers, Materials & Continua 2022, 73(2), 3685-3703. https://doi.org/10.32604/cmc.2022.026370
Received 24 December 2021; Accepted 18 April 2022; Issue published 16 June 2022
Abstract
As the Internet of Things (IoT) and mobile devices have rapidly proliferated, their computationally intensive applications have developed into complex, concurrent IoT-based workflows involving multiple interdependent tasks. By exploiting its low latency and high bandwidth, mobile edge computing (MEC) has emerged to achieve the high-performance computation offloading of these applications to satisfy the quality-of-service requirements of workflows and devices. In this study, we propose an offloading strategy for IoT-based workflows in a high-performance MEC environment. The proposed task-based offloading strategy consists of an optimization problem that includes task dependency, communication costs, workflow constraints, device energy consumption, and the heterogeneous characteristics of the edge environment. In addition, the optimal placement of workflow tasks is optimized using a discrete teaching learning-based optimization (DTLBO) metaheuristic. Extensive experimental evaluations demonstrate that the proposed offloading strategy is effective at minimizing the energy consumption of mobile devices and reducing the execution times of workflows compared to offloading strategies using different metaheuristics, including particle swarm optimization and ant colony optimization.Keywords
Cite This Article
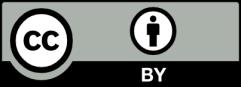