Open Access
ARTICLE
Gaussian Optimized Deep Learning-based Belief Classification Model for Breast Cancer Detection
1 Department of Industrial and Systems Engineering, College of Engineering, Princess Nourah Bint Abdulrahman University, P.O. Box 84428, Riyadh 11671, Saudi Arabia
2 Department of Biomedical Engineering, College of Engineering, Princess Nourah Bint Abdulrahman University, P.O. Box 84428, Riyadh, 11671, Saudi Arabia
3 Department of Computer Sciences, College of Computing and Information System, Umm Al-Qura University, Saudi Arabia
4 Department of Digital Media, Faculty of Computers and Information Technology, Future University in Egypt, New Cairo, 11845, Egypt
5 Department of Computer and Self Development, Preparatory Year Deanship, Prince Sattam Bin Abdulaziz University, AlKharj, Saudi Arabia
* Corresponding Author: Manar Ahmed Hamza. Email:
Computers, Materials & Continua 2022, 73(2), 4123-4138. https://doi.org/10.32604/cmc.2022.030492
Received 27 March 2022; Accepted 09 May 2022; Issue published 16 June 2022
Abstract
With the rapid increase of new cases with an increased mortality rate, cancer is considered the second and most deadly disease globally. Breast cancer is the most widely affected cancer worldwide, with an increased death rate percentage. Due to radiologists’ processing of mammogram images, many computer-aided diagnoses have been developed to detect breast cancer. Early detection of breast cancer will reduce the death rate worldwide. The early diagnosis of breast cancer using the developed computer-aided diagnosis (CAD) systems still needed to be enhanced by incorporating innovative deep learning technologies to improve the accuracy and sensitivity of the detection system with a reduced false positive rate. This paper proposed an efficient and optimized deep learning-based feature selection approach with this consideration. This model selects the relevant features from the mammogram images that can improve the accuracy of malignant detection and reduce the false alarm rate. Transfer learning is used in the extraction of features initially. Na ext, a convolution neural network, is used to extract the features. The two feature vectors are fused and optimized with enhanced Butterfly Optimization with Gaussian function (TL-CNN-EBOG) to select the final most relevant features. The optimized features are applied to the classifier called Deep belief network (DBN) to classify the benign and malignant images. The feature extraction and classification process used two datasets, breast, and MIAS. Compared to the existing methods, the optimized deep learning-based model secured 98.6% of improved accuracy on the breast dataset and 98.85% of improved accuracy on the MIAS dataset.Keywords
Cite This Article
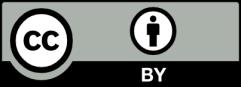