Open Access
ARTICLE
An Intelligent Hazardous Waste Detection and Classification Model Using Ensemble Learning Techniques
1 Department of Computer Science, College of Sciences and Humanities-Aflaj, Prince Sattam bin Abdulaziz University, Saudi Arabia
2 Department of Information Systems, College of Computing and Information System, Umm Al-Qura University, Saudi Arabia
3 Department of Information Systems, College of Computer and Information Sciences, Princess Nourah bint Abdulrahman University, P.O. Box 84428, Riyadh, 11671, Saudi Arabia
4 Department of Computer Science, College of Science and Arts at Muhayel, King Khalid University, Muhayel Aseer, Saudi Arabia
5 Department of Computer Science, Faculty of Computers and Information Technology, Future University in Egypt, New Cairo, 11835, Egypt
6 Department of Computer and Self Development, Preparatory Year Deanship, Prince Sattam bin Abdulaziz University, AlKharj, Saudi Arabia
* Corresponding Author: Mesfer Al Duhayyim. Email:
Computers, Materials & Continua 2023, 74(2), 3315-3332. https://doi.org/10.32604/cmc.2023.033250
Received 12 June 2022; Accepted 09 August 2022; Issue published 31 October 2022
Abstract
Proper waste management models using recent technologies like computer vision, machine learning (ML), and deep learning (DL) are needed to effectively handle the massive quantity of increasing waste. Therefore, waste classification becomes a crucial topic which helps to categorize waste into hazardous or non-hazardous ones and thereby assist in the decision making of the waste management process. This study concentrates on the design of hazardous waste detection and classification using ensemble learning (HWDC-EL) technique to reduce toxicity and improve human health. The goal of the HWDC-EL technique is to detect the multiple classes of wastes, particularly hazardous and non-hazardous wastes. The HWDC-EL technique involves the ensemble of three feature extractors using Model Averaging technique namely discrete local binary patterns (DLBP), EfficientNet, and DenseNet121. In addition, the flower pollination algorithm (FPA) based hyperparameter optimizers are used to optimally adjust the parameters involved in the EfficientNet and DenseNet121 models. Moreover, a weighted voting-based ensemble classifier is derived using three machine learning algorithms namely support vector machine (SVM), extreme learning machine (ELM), and gradient boosting tree (GBT). The performance of the HWDC-EL technique is tested using a benchmark Garbage dataset and it obtains a maximum accuracy of 98.85%.Keywords
Cite This Article
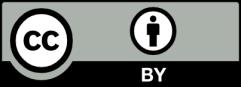