Open Access
ARTICLE
Two-Stream Deep Learning Architecture-Based Human Action Recognition
1 Department of Computer Science, COMSATS University Islamabad, Wah Campus, Pakistan
2 Department of Computer Science, HITEC University, Taxila, Pakistan
3 Department of Computer Science, Bahria University, Islamabad, Pakistan
4 Computer Sciences Department, College of Computer and Information Sciences, Princess Nourah bint Abdulrahman University, Riyadh, 11671, Saudi Arabia
5 College of Computer Engineering and Science, Prince Sattam Bin Abdulaziz University, Al-Kharaj, 11942, Saudi Arabia
6 Faculty of Engineering, Imperial College London, London, SW7 2AZ, UK
7 College of Arts, Media, and Technology, Chiang Mai University, Chiang Mai, 50200, Thailand
* Corresponding Author: Orawit Thinnukool. Email:
Computers, Materials & Continua 2023, 74(3), 5931-5949. https://doi.org/10.32604/cmc.2023.028743
Received 16 February 2022; Accepted 06 May 2022; Issue published 28 December 2022
Abstract
Human action recognition (HAR) based on Artificial intelligence reasoning is the most important research area in computer vision. Big breakthroughs in this field have been observed in the last few years; additionally, the interest in research in this field is evolving, such as understanding of actions and scenes, studying human joints, and human posture recognition. Many HAR techniques are introduced in the literature. Nonetheless, the challenge of redundant and irrelevant features reduces recognition accuracy. They also faced a few other challenges, such as differing perspectives, environmental conditions, and temporal variations, among others. In this work, a deep learning and improved whale optimization algorithm based framework is proposed for HAR. The proposed framework consists of a few core stages i.e., frames initial preprocessing, fine-tuned pre-trained deep learning models through transfer learning (TL), features fusion using modified serial based approach, and improved whale optimization based best features selection for final classification. Two pre-trained deep learning models such as InceptionV3 and Resnet101 are fine-tuned and TL is employed to train on action recognition datasets. The fusion process increases the length of feature vectors; therefore, improved whale optimization algorithm is proposed and selects the best features. The best selected features are finally classified using machine learning (ML) classifiers. Four publicly accessible datasets such as Ut-interaction, Hollywood, Free Viewpoint Action Recognition using Motion History Volumes (IXMAS), and centre of computer vision (UCF) Sports, are employed and achieved the testing accuracy of 100%, 99.9%, 99.1%, and 100% respectively. Comparison with state of the art techniques (SOTA), the proposed method showed the improved accuracy.Keywords
Cite This Article
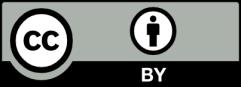