Open Access
ARTICLE
Sine Cosine Optimization with Deep Learning-Based Applied Linguistics for Sentiment Analysis on COVID-19 Tweets
1 Department of Computer and Self Development, Preparatory Year Deanship, Prince Sattam bin Abdulaziz University, AlKharj, Saudi Arabia
2 Department of Applied Linguistics, College of Languages, Princess Nourah bint Abdulrahman University, P.O. Box 84428, Riyadh, 11671, Saudi Arabia
3 Department of English, College of Science and Arts at Mahayil, King Khalid University, Muhayil, 63763, Saudi Arabia
4 Department of Computer Sciences, College of Computing and Information System, Umm Al-Qura University, Makkah, 24211, Saudi Arabia
5 Department of Digital Media, Faculty of Computers and Information Technology, Future University in Egypt,New Cairo, 11845, Egypt
6 Department of English, College of Science & Humanities, Prince Sattam bin Abdulaziz University, AlKharj, Saudi Arabia
* Corresponding Author: Abdelwahed Motwakel. Email:
Computers, Materials & Continua 2023, 75(3), 4767-4783. https://doi.org/10.32604/cmc.2023.034840
Received 29 July 2022; Accepted 15 November 2022; Issue published 29 April 2023
Abstract
Applied linguistics is an interdisciplinary domain which identifies, investigates, and offers solutions to language-related real-life problems. The new coronavirus disease, otherwise known as Coronavirus disease (COVID-19), has severely affected the everyday life of people all over the world. Specifically, since there is insufficient access to vaccines and no straight or reliable treatment for coronavirus infection, the country has initiated the appropriate preventive measures (like lockdown, physical separation, and masking) for combating this extremely transmittable disease. So, individuals spent more time on online social media platforms (i.e., Twitter, Facebook, Instagram, LinkedIn, and Reddit) and expressed their thoughts and feelings about coronavirus infection. Twitter has become one of the popular social media platforms and allows anyone to post tweets. This study proposes a sine cosine optimization with bidirectional gated recurrent unit-based sentiment analysis (SCOBGRU-SA) on COVID-19 tweets. The SCOBGRU-SA technique aimed to detect and classify the various sentiments in Twitter data during the COVID-19 pandemic. The SCOBGRU-SA technique follows data pre-processing and the Fast-Text word embedding process to accomplish this. Moreover, the BGRU model is utilized to recognise and classify sentiments present in the tweets. Furthermore, the SCO algorithm is exploited for tuning the BGRU method’s hyperparameter, which helps attain improved classification performance. The experimental validation of the SCOBGRU-SA technique takes place using a benchmark dataset, and the results signify its promising performance compared to other DL models.Keywords
Cite This Article
A. Motwakel, H. J. Alshahrani, A. Q. A. Hassan, K. Tarmissi, A. S. Mehanna et al., "Sine cosine optimization with deep learning-based applied linguistics for sentiment analysis on covid-19 tweets," Computers, Materials & Continua, vol. 75, no.3, pp. 4767–4783, 2023. https://doi.org/10.32604/cmc.2023.034840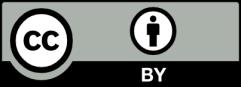