Open Access
ARTICLE
Supervised Feature Learning for Offline Writer Identification Using VLAD and Double Power Normalization
1 College of Computer, Nanjing University of Posts and Telecommunications, Nanjing, 210023, China
2 Department of Computer Information and Cyber Security, Jiangsu Police Institute, Nanjing, 210031, China
3 JinCheng College, Nanjing University of Aeronautics and Astronautics, Nanjing, 211156, China
4 Engineering Research Center of Electronic Data Forensics Analysis, Nanjing, 210031, Jiangsu Province, China
* Corresponding Author: Meng Wu. Email:
Computers, Materials & Continua 2023, 76(1), 279-293. https://doi.org/10.32604/cmc.2023.035279
Received 15 August 2022; Accepted 08 February 2023; Issue published 08 June 2023
Abstract
As an indispensable part of identity authentication, offline writer identification plays a notable role in biology, forensics, and historical document analysis. However, identifying handwriting efficiently, stably, and quickly is still challenging due to the method of extracting and processing handwriting features. In this paper, we propose an efficient system to identify writers through handwritten images, which integrates local and global features from similar handwritten images. The local features are modeled by effective aggregate processing, and global features are extracted through transfer learning. Specifically, the proposed system employs a pre-trained Residual Network to mine the relationship between large image sets and specific handwritten images, while the vector of locally aggregated descriptors with double power normalization is employed in aggregating local and global features. Moreover, handwritten image segmentation, preprocessing, enhancement, optimization of neural network architecture, and normalization for local and global features are exploited, significantly improving system performance. The proposed system is evaluated on Computer Vision Lab (CVL) datasets and the International Conference on Document Analysis and Recognition (ICDAR) 2013 datasets. The results show that it represents good generalizability and achieves state-of-the-art performance. Furthermore, the system performs better when training complete handwriting patches with the normalization method. The experimental result indicates that it’s significant to segment handwriting reasonably while dealing with handwriting overlap, which reduces visual burstiness.Keywords
Cite This Article
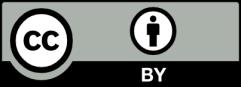