Open Access
ARTICLE
Text Augmentation-Based Model for Emotion Recognition Using Transformers
1 Department of Computer Science, The University of Haripur, Haripur, 22620, Pakistan
2 Department of Computer Systems Engineering, Faculty of Electrical and Computer Engineering, University of Engineering and Technology Peshawar, Peshawar, 25120, Pakistan
3 Department of Electronics Engineering Technology, University of Technology, Nowshera, 24100, Pakistan
4 Department of Computer Science, Shaheed Benazir Bhutto Women University, Peshawar, 25000, Pakistan
5 Department of Information Systems, College of Computer and Information Sciences, Princess Nourah bint Abdulrahman University, Riyadh, 11671, Saudi Arabia
* Corresponding Author: Fida Mohammad. Email:
(This article belongs to the Special Issue: Advance Machine Learning for Sentiment Analysis over Various Domains and Applications)
Computers, Materials & Continua 2023, 76(3), 3523-3547. https://doi.org/10.32604/cmc.2023.040202
Received 08 March 2023; Accepted 04 July 2023; Issue published 08 October 2023
Abstract
Emotion Recognition in Conversations (ERC) is fundamental in creating emotionally intelligent machines. Graph-Based Network (GBN) models have gained popularity in detecting conversational contexts for ERC tasks. However, their limited ability to collect and acquire contextual information hinders their effectiveness. We propose a Text Augmentation-based computational model for recognizing emotions using transformers (TA-MERT) to address this. The proposed model uses the Multimodal Emotion Lines Dataset (MELD), which ensures a balanced representation for recognizing human emotions. The model used text augmentation techniques to produce more training data, improving the proposed model’s accuracy. Transformer encoders train the deep neural network (DNN) model, especially Bidirectional Encoder (BE) representations that capture both forward and backward contextual information. This integration improves the accuracy and robustness of the proposed model. Furthermore, we present a method for balancing the training dataset by creating enhanced samples from the original dataset. By balancing the dataset across all emotion categories, we can lessen the adverse effects of data imbalance on the accuracy of the proposed model. Experimental results on the MELD dataset show that TA-MERT outperforms earlier methods, achieving a weighted F1 score of 62.60% and an accuracy of 64.36%. Overall, the proposed TA-MERT model solves the GBN models’ weaknesses in obtaining contextual data for ERC. TA-MERT model recognizes human emotions more accurately by employing text augmentation and transformer-based encoding. The balanced dataset and the additional training samples also enhance its resilience. These findings highlight the significance of transformer-based approaches for special emotion recognition in conversations.Keywords
Cite This Article
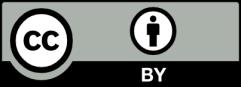