Open Access
ARTICLE
Push-Based Content Dissemination and Machine Learning-Oriented Illusion Attack Detection in Vehicular Named Data Networking
1 State Key Laboratory of Networking and Switching Technology, Beijing University of Post and Telecommunication, Beijing, 100876, China
2 Department of Computer Engineering, College of Computer and Information Sciences, King Saud University, Riyadh, 11543, Saudi Arabia
3 School of Computer Science and Technology, Harbin Institute of Technology, Harbin, 150001, China
4 School of Computer Science and Electronic Engineering, University of Essex, Colchester, CO4 3SQ, UK
* Corresponding Author: Ghulam Muhammad. Email:
(This article belongs to the Special Issue: Advance Machine Learning for Sentiment Analysis over Various Domains and Applications)
Computers, Materials & Continua 2023, 76(3), 3131-3150. https://doi.org/10.32604/cmc.2023.040784
Received 03 March 2023; Accepted 21 June 2023; Issue published 08 October 2023
Abstract
Recent advancements in the Vehicular Ad-hoc Network (VANET) have tremendously addressed road-related challenges. Specifically, Named Data Networking (NDN) in VANET has emerged as a vital technology due to its outstanding features. However, the NDN communication framework fails to address two important issues. The current NDN employs a pull-based content retrieval network, which is inefficient in disseminating crucial content in Vehicular Named Data Networking (VNDN). Additionally, VNDN is vulnerable to illusion attackers due to the administrative-less network of autonomous vehicles. Although various solutions have been proposed for detecting vehicles’ behavior, they inadequately addressed the challenges specific to VNDN. To deal with these two issues, we propose a novel push-based crucial content dissemination scheme that extends the scope of VNDN from pull-based content retrieval to a push-based content forwarding mechanism. In addition, we exploit Machine Learning (ML) techniques within VNDN to detect the behavior of vehicles and classify them as attackers or legitimate. We trained and tested our system on the publicly accessible dataset Vehicular Reference Misbehavior (VeReMi). We employed five ML classification algorithms and constructed the best model for illusion attack detection. Our results indicate that Random Forest (RF) achieved excellent accuracy in detecting all illusion attack types in VeReMi, with an accuracy rate of 100% for type 1 and type 2, 96% for type 4 and type 16, and 95% for type 8. Thus, RF can effectively evaluate the behavior of vehicles and identify attacker vehicles with high accuracy. The ultimate goal of our research is to improve content exchange and secure VNDN from attackers. Thus, our ML-based attack detection and prevention mechanism ensures trustworthy content dissemination and prevents attacker vehicles from sharing misleading information in VNDN.Keywords
Cite This Article
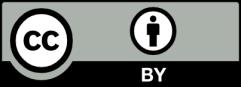