Open Access
ARTICLE
Clinical Knowledge-Based Hybrid Swin Transformer for Brain Tumor Segmentation
1 College of Information Science and Engineering, Northeastern University, Shenyang, 110819, China
2 Faculty of Robot Science and Engineer, Northeastern University, Shenyang, 110819, China
3 Faculty of Engineering, Macquarie University, Sydney, NSW, 2109, Australia
* Corresponding Authors: Xiaosheng Yu. Email: ; Chengdong Wu. Email:
Computers, Materials & Continua 2023, 76(3), 3797-3811. https://doi.org/10.32604/cmc.2023.042069
Received 17 May 2023; Accepted 25 July 2023; Issue published 08 October 2023
Abstract
Accurate tumor segmentation from brain tissues in Magnetic Resonance Imaging (MRI) imaging is crucial in the pre-surgical planning of brain tumor malignancy. MRI images’ heterogeneous intensity and fuzzy boundaries make brain tumor segmentation challenging. Furthermore, recent studies have yet to fully employ MRI sequences’ considerable and supplementary information, which offers critical a priori knowledge. This paper proposes a clinical knowledge-based hybrid Swin Transformer multimodal brain tumor segmentation algorithm based on how experts identify malignancies from MRI images. During the encoder phase, a dual backbone network with a Swin Transformer backbone to capture long dependencies from 3D MR images and a Convolutional Neural Network (CNN)-based backbone to represent local features have been constructed. Instead of directly connecting all the MRI sequences, the proposed method re-organizes them and splits them into two groups based on MRI principles and characteristics: T1 and T1ce, T2 and Flair. These aggregated images are received by the dual-stem Swin Transformer-based encoder branch, and the multimodal sequence-interacted cross-attention module (MScAM) captures the interactive information between two sets of linked modalities in each stage. In the CNN-based encoder branch, a triple down-sampling module (TDsM) has been proposed to balance the performance while downsampling. In the final stage of the encoder, the feature maps acquired from two branches are concatenated as input to the decoder, which is constrained by MScAM outputs. The proposed method has been evaluated on datasets from the MICCAI BraTS2021 Challenge. The results of the experiments demonstrate that the method algorithm can precisely segment brain tumors, especially the portions within tumors.Keywords
Cite This Article
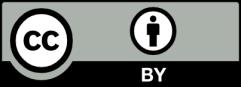