Open Access
ARTICLE
RF-Net: Unsupervised Low-Light Image Enhancement Based on Retinex and Exposure Fusion
College of Computer Science and Technology, Xi’an University of Science and Technology, Xi’an, 710054, China
* Corresponding Author: Chenhui Fu. Email:
(This article belongs to the Special Issue: Advances and Applications in Signal, Image and Video Processing)
Computers, Materials & Continua 2023, 77(1), 1103-1122. https://doi.org/10.32604/cmc.2023.042416
Received 29 May 2023; Accepted 25 August 2023; Issue published 31 October 2023
Abstract
Low-light image enhancement methods have limitations in addressing issues such as color distortion, lack of vibrancy, and uneven light distribution and often require paired training data. To address these issues, we propose a two-stage unsupervised low-light image enhancement algorithm called Retinex and Exposure Fusion Network (RF-Net), which can overcome the problems of over-enhancement of the high dynamic range and under-enhancement of the low dynamic range in existing enhancement algorithms. This algorithm can better manage the challenges brought about by complex environments in real-world scenarios by training with unpaired low-light images and regular-light images. In the first stage, we design a multi-scale feature extraction module based on Retinex theory, capable of extracting details and structural information at different scales to generate high-quality illumination and reflection images. In the second stage, an exposure image generator is designed through the camera response mechanism function to acquire exposure images containing more dark features, and the generated images are fused with the original input images to complete the low-light image enhancement. Experiments show the effectiveness and rationality of each module designed in this paper. And the method reconstructs the details of contrast and color distribution, outperforms the current state-of-the-art methods in both qualitative and quantitative metrics, and shows excellent performance in the real world.Keywords
Cite This Article
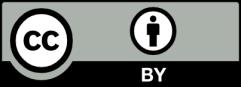