Open Access
ARTICLE
Adaptive Deep Learning Model to Enhance Smart Greenhouse Agriculture
1
Department of Computer Science, College of Computer and Information Sciences, Jouf University, P.O. Box 72314,
Skaka, Saudi Arabia
2
Department of Computer Science, Faculty of Computers and Information, Menoufia University, P.O. Box 6121890, Menoufia,
Egypt
3
Department of Software Engineering, College of Computer and Information Sciences, Jouf University, P.O. Box 72314, Skaka,
Saudi Arabia
4
RIADI Laboratory, La Manouba University, P.O. Box, 2010, Manouba, Tunisia
5
Department of Information Systems, College of Computer and Information Sciences, Princess Nourah bint Abdulrahman
University, P.O. Box 84428, Riyadh, 11671, Saudi Arabia
6
Department of Information Systems and Technology, Faculty of Graduate Studies and Research, Cairo University, P.O. Box
3753450, Giza, Egypt
* Corresponding Author: Leila Jamel. Email:
Computers, Materials & Continua 2023, 77(2), 2545-2564. https://doi.org/10.32604/cmc.2023.042179
Received 21 May 2023; Accepted 04 September 2023; Issue published 29 November 2023
Abstract
The trend towards smart greenhouses stems from various factors, including a lack of agricultural land area owing to population concentration and housing construction on agricultural land, as well as water shortages. This study proposes building a full farming adaptation model that depends on current sensor readings and available datasets from different agricultural research centers. The proposed model uses a one-dimensional convolutional neural network (CNN) deep learning model to control the growth of strategic crops, including cucumber, pepper, tomato, and bean. The proposed model uses the Internet of Things (IoT) to collect data on agricultural operations and then uses this data to control and monitor these operations in real time. This helps to ensure that crops are getting the right amount of fertilizer, water, light, and temperature, which can lead to improved yields and a reduced risk of crop failure. Our dataset is based on data collected from expert farmers, the photovoltaic construction process, agricultural engineers, and research centers. The experimental results showed that the precision, recall, F1-measures, and accuracy of the one-dimensional CNN for the tested dataset were approximately 97.3%, 98.2%, 97.25%, and 97.56%, respectively. The new smart greenhouse automation system was also evaluated on four crops with a high turnover rate. The system has been found to be highly effective in terms of crop productivity, temperature management and water conservation.Keywords
Cite This Article
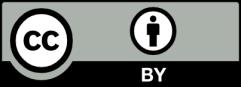