Open Access
ARTICLE
An Enhanced Equilibrium Optimizer for Solving Optimization Tasks
1 School of Information Science and Engineering, Yunnan University, Kunming, 650500, China
2 Department of Electrical and Computer Engineering, Lebanese American University, Byblos, 1401, Lebanon
3 University Centre for Research and Development, Department of Computer Science and Engineering, Chandigarh University, Gharuan, Mohali, 140413, India
4 Department of Computer Science and Engineering, Graphic Era (Deemed to be University), Dehradun, 248002, India
5 Research and Development Department, Youbei Technology Co., Ltd., Kunming, 650500, China
* Corresponding Authors: Hongwei Ding. Email: ; Zongshan Wang. Email:
(This article belongs to the Special Issue: Optimization for Artificial Intelligence Application)
Computers, Materials & Continua 2023, 77(2), 2385-2406. https://doi.org/10.32604/cmc.2023.039883
Received 22 February 2023; Accepted 11 August 2023; Issue published 29 November 2023
Abstract
The equilibrium optimizer (EO) represents a new, physics-inspired metaheuristic optimization approach that draws inspiration from the principles governing the control of volume-based mixing to achieve dynamic mass equilibrium. Despite its innovative foundation, the EO exhibits certain limitations, including imbalances between exploration and exploitation, the tendency to local optima, and the susceptibility to loss of population diversity. To alleviate these drawbacks, this paper introduces an improved EO that adopts three strategies: adaptive inertia weight, Cauchy mutation, and adaptive sine cosine mechanism, called SCEO. Firstly, a new update formula is conceived by incorporating an adaptive inertia weight to reach an appropriate balance between exploration and exploitation. Next, an adaptive sine cosine mechanism is embedded to boost the global exploratory capacity. Finally, the Cauchy mutation is utilized to prevent the loss of population diversity during searching. To validate the efficacy of the proposed SCEO, a comprehensive evaluation is conducted on 15 classical benchmark functions and the CEC2017 test suite. The outcomes are subsequently benchmarked against both the conventional EO, its variants, and other cutting-edge metaheuristic techniques. The comparisons reveal that the SCEO method provides significantly superior results against the standard EO and other competitors. In addition, the developed SCEO is implemented to deal with a mobile robot path planning (MRPP) task, and compared to some classical metaheuristic approaches. The analysis results demonstrate that the SCEO approach provides the best performance and is a prospective tool for MRPP.Keywords
Cite This Article
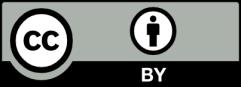