Open Access
ARTICLE
Electromyogram Based Personal Recognition Using Attention Mechanism for IoT Security
IT Research Institute, Chosun University, Gwang-Ju, 61452, Korea
* Corresponding Author: Sungbum Pan. Email:
(This article belongs to the Special Issue: Innovative Security for the Next Generation Mobile Communication and Internet Systems)
Computers, Materials & Continua 2023, 77(2), 1663-1678. https://doi.org/10.32604/cmc.2023.043998
Received 18 July 2023; Accepted 15 September 2023; Issue published 29 November 2023
Abstract
As Internet of Things (IoT) technology develops, integrating network functions into diverse equipment introduces new challenges, particularly in dealing with counterfeit issues. Over the past few decades, research efforts have focused on leveraging electromyogram (EMG) for personal recognition, aiming to address security concerns. However, obtaining consistent EMG signals from the same individual is inherently challenging, resulting in data irregularity issues and consequently decreasing the accuracy of personal recognition. Notably, conventional studies in EMG-based personal recognition have overlooked the issue of data irregularities. This paper proposes an innovative approach to personal recognition that combines a siamese fusion network with an auxiliary classifier, effectively mitigating the impact of data irregularities in EMG-based recognition. The proposed method employs empirical mode decomposition (EMD) to extract distinctive features. The model comprises two sub-networks designed to follow the siamese network architecture and a decision network integrated with the novel auxiliary classifier, specifically designed to address data irregularities. The two sub-networks sharing a weight calculate the compatibility function. The auxiliary classifier collaborates with a neural network to implement an attention mechanism. The attention mechanism using the auxiliary classifier solves the data irregularity problem by improving the importance of the EMG gesture section. Experimental results validated the efficacy of the proposed personal recognition method, achieving a remarkable 94.35% accuracy involving 100 subjects from the multi-session CU_sEMG database (DB). This performance outperforms the existing approaches by 3%, employing auxiliary classifiers. Furthermore, an additional experiment yielded an improvement of over 0.85% of Ninapro DB, 3% of CU_sEMG DB compared to the existing EMG-based recognition methods. Consequently, the proposed personal recognition using EMG proves to secure IoT devices, offering robustness against data irregularities.Keywords
Cite This Article
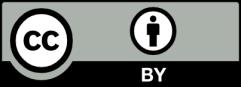