Open Access
ARTICLE
Zero-DCE++ Inspired Object Detection in Less Illuminated Environment Using Improved YOLOv5
1 Centre for Cyber Physical Systems, Vellore Institute of Technology (VIT), Chennai, Tamil Nadu, 600127, India
2 School of Computer Science and Engineering, Vellore Institute of Technology (VIT), Chennai, Tamil Nadu, 600127, India
3 School of Information and Data Sciences, Nagasaki University, Nagasaki, 8528521, Japan
* Corresponding Author: Ananthakrishnan Balasundaram. Email:
(This article belongs to the Special Issue: Deep Learning based Object Detection and Tracking in Videos)
Computers, Materials & Continua 2023, 77(3), 2751-2769. https://doi.org/10.32604/cmc.2023.044374
Received 28 July 2023; Accepted 07 November 2023; Issue published 26 December 2023
Abstract
Automated object detection has received the most attention over the years. Use cases ranging from autonomous driving applications to military surveillance systems, require robust detection of objects in different illumination conditions. State-of-the-art object detectors tend to fare well in object detection during daytime conditions. However, their performance is severely hampered in night light conditions due to poor illumination. To address this challenge, the manuscript proposes an improved YOLOv5-based object detection framework for effective detection in unevenly illuminated nighttime conditions. Firstly, the preprocessing strategies involve using the Zero-DCE++ approach to enhance lowlight images. It is followed by optimizing the existing YOLOv5 architecture by integrating the Convolutional Block Attention Module (CBAM) in the backbone network to boost model learning capability and Depthwise Convolutional module (DWConv) in the neck network for efficient compression of network parameters. The Night Object Detection (NOD) and Exclusively Dark (ExDARK) dataset has been used for this work. The proposed framework detects classes like humans, bicycles, and cars. Experiments demonstrate that the proposed architecture achieved a higher Mean Average Precision (mAP) along with a reduction in model size and total parameters, respectively. The proposed model is lighter by 11.24% in terms of model size and 12.38% in terms of parameters when compared to baseline YOLOv5.Keywords
Cite This Article
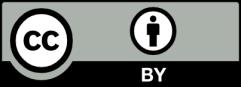