Open Access
ARTICLE
Maximizing Influence in Temporal Social Networks: A Node Feature-Aware Voting Algorithm
1 College of Computer and Control Engineering, Qiqihar University, Qiqihar, 161006, China
2 Heilongjiang Key Laboratory of Big Data Network Security Detection and Analysis, Qiqihar University, Qiqihar, 161006, China
3 College of Teacher Education, Qiqihar University, Qiqihar, 161006, China
* Corresponding Author: Wenlong Zhu. Email:
Computers, Materials & Continua 2023, 77(3), 3095-3117. https://doi.org/10.32604/cmc.2023.045646
Received 03 September 2023; Accepted 27 October 2023; Issue published 26 December 2023
Abstract
Influence Maximization (IM) aims to select a seed set of size k in a social network so that information can be spread most widely under a specific information propagation model through this set of nodes. However, most existing studies on the IM problem focus on static social network features, while neglecting the features of temporal social networks. To bridge this gap, we focus on node features reflected by their historical interaction behavior in temporal social networks, i.e., interaction attributes and self-similarity, and incorporate them into the influence maximization algorithm and information propagation model. Firstly, we propose a node feature-aware voting algorithm, called ISVoteRank, for seed nodes selection. Specifically, before voting, the algorithm sets the initial voting ability of nodes in a personalized manner by combining their features. During the voting process, voting weights are set based on the interaction strength between nodes, allowing nodes to vote at different extents and subsequently weakening their voting ability accordingly. The process concludes by selecting the top k nodes with the highest voting scores as seeds, avoiding the inefficiency of iterative seed selection in traditional voting-based algorithms. Secondly, we extend the Independent Cascade (IC) model and propose the Dynamic Independent Cascade (DIC) model, which aims to capture the dynamic features in the information propagation process by combining node features. Finally, experiments demonstrate that the ISVoteRank algorithm has been improved in both effectiveness and efficiency compared to baseline methods, and the influence spread through the DIC model is improved compared to the IC model.Keywords
Cite This Article
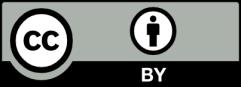