Open Access
ARTICLE
Augmented Deep Multi-Granularity Pose-Aware Feature Fusion Network for Visible-Infrared Person Re-Identification
School of Educational Science and Technology, Nanjing University of Posts and Telecommunications, Nanjing, 210013, China
* Corresponding Author: Wanru Song. Email:
Computers, Materials & Continua 2023, 77(3), 3467-3488. https://doi.org/10.32604/cmc.2023.045849
Received 09 September 2023; Accepted 07 November 2023; Issue published 26 December 2023
Abstract
Visible-infrared Cross-modality Person Re-identification (VI-ReID) is a critical technology in smart public facilities such as cities, campuses and libraries. It aims to match pedestrians in visible light and infrared images for video surveillance, which poses a challenge in exploring cross-modal shared information accurately and efficiently. Therefore, multi-granularity feature learning methods have been applied in VI-ReID to extract potential multi-granularity semantic information related to pedestrian body structure attributes. However, existing research mainly uses traditional dual-stream fusion networks and overlooks the core of cross-modal learning networks, the fusion module. This paper introduces a novel network called the Augmented Deep Multi-Granularity Pose-Aware Feature Fusion Network (ADMPFF-Net), incorporating the Multi-Granularity Pose-Aware Feature Fusion (MPFF) module to generate discriminative representations. MPFF efficiently explores and learns global and local features with multi-level semantic information by inserting disentangling and duplicating blocks into the fusion module of the backbone network. ADMPFF-Net also provides a new perspective for designing multi-granularity learning networks. By incorporating the multi-granularity feature disentanglement (mGFD) and posture information segmentation (pIS) strategies, it extracts more representative features concerning body structure information. The Local Information Enhancement (LIE) module augments high-performance features in VI-ReID, and the multi-granularity joint loss supervises model training for objective feature learning. Experimental results on two public datasets show that ADMPFF-Net efficiently constructs pedestrian feature representations and enhances the accuracy of VI-ReID.Keywords
Person re-identification (Person ReID) aims to retrieve the same target pedestrian from various cameras with non-overlapping viewpoints. With the popularization of surveillance devices and the continuous development of computer vision, ReID has received increasing academic attention. As methods based on deep learning methods are continuously proposed, the performance of the ReID task has been highly improved [1–3]. However, in the real-world application of surveillance, there are frequently low-light situations, such as at night. With the popularity of 24-h surveillance cameras, those in public places can switch to infrared mode by themselves at night. For another example, with the increasing significance of big data and artificial intelligence technology, there is a growing emphasis on providing students who will inhabit the future society with education in data science and AI [4]. In the process of building a teaching environment such as a Digital Campus, the infrared camera can be added to the surveillance system to ensure the security of the place and the diversity of educational information. It seems to be a challenging issue if the retrieval of pedestrians or students is performed in the above application scenarios. Additionally, Internet of Things (IoT) based interconnected vision sensors in smart cities are considered essential elements of a cyber-physical system (CPS) and contribute significantly to urban security. However, the Re-ID of targeted persons using emerging edge AI techniques still faces certain challenges [5]. Consequently, the visible-infrared cross-modality person re-identification (VI-ReID) has also emerged.
In the visible-visible ReID (VV-ReID) task, researchers need to deal with the discrepancies between pedestrian images of the same identity (ID) to achieve accurate recognition. The discrepancies can be regarded as intra-modality differences, which are generated by changes in pose, environment, etc. The network design [6–8] and the distance metric loss [9,10] are utilized to narrow the distance of the same ID and enlarge the intra-class differences of different pedestrians. In contrast to VV-ReID, a significant and central challenge in VI-ReID technology lies in addressing the substantial inter-class differences caused by the modality variations between visible and infrared images. The visible and infrared images are respectively captured by reflected light and thermal radiation. Hence, there is an inherent difference between the imaging principles of both, resulting in captured images of pedestrians presenting diverse appearances in these two domains. In addition, the problems due to the short development time and the methods mostly migrated from the traditional VV-ReID are still preventing further in-depth development and research.
In order to achieve the accuracy improvement of VI-ReID, researchers have investigated three aspects of shared feature mining [11–15]: heterogeneous distance loss function design [16–19] and heterogeneous image Translation [20–23]. For feature learning, existing methods [11,16,24] generally perform coarse-grained learning from the image-level global feature. Although they can reduce the inter-modal disparity, the fine-grained representation of detailed information and body structure of pedestrians represented by local features are neglected to a larger extent. Several works [17,25,26] focused on more detailed local features through methods based on slicing, such as PCB. These methods bridge the gap between a great quantity of useful fine-grained information in cross-modal tasks. Existing approaches of local feature extraction are mostly based on average segmentation of the feature map that is outputted from the backbone. However, after extensive and detailed observation of the dataset, we also realize that it is difficult for the equal-scaled slices to describe the body structure accurately. This leads to high redundancy and low accuracy of the obtained fine-grained information. In fact, it is due to body misalignment caused by the different shooting perspectives. Moreover, it brings another problem simultaneously: excessive attention to local features may inevitably result in ignoring coarse-grained information about pedestrians, such as posture and body shape.
Multiple Granularities Network (MGN) certainly provides a great solution to this issue [27,28]. It integrates various detailed information with coarse-grained. Authors explore and improve MGN for efficient supervised learning of multiple granularity information. However, the network structure proposed in [28] is much more complicated and redundant. It is a four-stream parallel learning by two branches, namely global and local branches, so as to realize the common attention to coarse and fine granularity. In turn, this leads to the issues of huge parameters and high computational costs. The existing MGN models are all derived from ReID, while the main difference in VI-ReID is the shared feature learning process across modalities. Therefore, constructing a shared network that allows the fusion module responsible for shared learning to transmit coarse-grained and fine-grained information more efficiently and deeply becomes the key to breakthroughs in future multi-granularity cross-modal ReID research.
To address the above issues and improve the effectiveness of the feature learning in the VI-ReID task, this paper proposes a novel framework named Augmented Deep Multi-granularity Pose-aware Feature Fusion Network (ADMPFF-Net) for mining the rich cross-modality information. ADMPFF-Net is designed based on the parameter-sharing network to learn global-local features simultaneously [17]. The network aims to learn multi-level shared features from pedestrians in the cross-modality task. To enable the fusion component, which serves as the core of the VI-ReID network, to learn multi-granularity information more deeply and comprehensively. The network utilizes the Multiple-granularity Pose-aware Feature Fusion (MPFF) module. The most significant difference between the Multiple-granularity Pose-aware Feature Fusion (MPFF) module and other multi-granularity feature fusion networks lies in its design approach. Traditional network designs often use a single fused module to separately extract coarse-grained and fine-grained features. In contrast, the MPFF module splits the fusion module into two parts: the first part continues to use a parameter-sharing network to fuse features, while the second part is duplicated and divided into multiple feature extraction units to achieve multi-granularity learning. This design approach enables the fusion module, which serves as the backbone of the network, to more effectively conduct deeper exploration and learn potential multi-granularity features. Additionally, this network proposes an improved slicing strategy based on the common characteristics of the body for fine-grained feature learning. This strategy chooses to use body-aware non-equal slices, thus better preserving better preserve the original body structure of humans and reducing the information redundancy caused by structural confusion. This strategy opts for non-uniform slicing based on pedestrian perception, dividing local features according to a 1:2:3 ratio for the head, upper body, and lower body. In contrast to traditional three equal parts slicing, it better preserves the original structure of the pedestrian body and reduces information redundancy caused by structural ambiguity. As a pioneering attempt in local feature extraction, its lightweight and efficient nature offers potential improvements for other methods and networks aimed at extracting local features. In order to achieve the optimization of the features that have a strong representation capability in the fine-grained information, the network is designed to replace the coarse-grained part of the global feature representation with the proposed local information enhancement (LIE) module. As a result, discrepancies among multi-granularity information can be compensated, and the effectiveness of particular feature representations can be enhanced. Thus, the accuracy of the network for multi-granularity information learning can be improved. Moreover, in order to supervise the learning process of multi-granularity features, we design a multi-granularity joint (MGJ) loss. The model’s loss is based on both traditional classification loss and triplet loss, enhancing its robustness.
In a nutshell, the contributions of this paper are summarized as follows:
• This paper proposes ADMPFF-Net for simultaneously learning multi-level features for VI-ReID. By deeply integrating multiple granularity units into a shared feature module, the model learns more robust representations in the cross-modality situation under the supervision of the MGJ loss.
• MPFF provides a novel and efficient backbone type for multi-granularity feature learning methods. Furthermore, combined with the innovative non-equal slicing strategy, it not only achieves precise and deep mining of multi-granularity features but also offers a promising avenue for related networks focused on local feature learning.
• LIE is designed to replace the global feature independent learning branch. It augments the learning of stable local features and solves the issue of model oscillations caused by different information scales.
• Extensive experiments are conducted on the two public datasets, and the results validate that the proposed method can achieve state-of-the-art performance on both datasets.
The rest of this paper is organized as follows. Section 2 discusses the work of VI-ReID related to this paper, and Section 3 gives an introduction to ADMPFF-Net in detail. To demonstrate the effectiveness of the proposed network, we present numerous experiments in Section 4, including both comparison experiments and ablation experiments. And finally, we bring a conclusion to the method in Section 5.
This section provides an overview of VI-ReID methods. The fundamental goal of all these methods is to minimize cross-modal discrepancies to the greatest extent possible while enhancing intra-class differences. Currently, the studies in the field of VI-ReID can be roughly divided into feature learning, metric learning, and image translation [2,3]. In particular, multi-granularity shared feature mining has been valued in the VI-ReID task as a new research direction in feature representation.
The essence of feature learning is to obtain more effective and robust representations of pedestrians in the cross-modal task. This approach primarily focuses on designing efficient network structures to learn and extract shared feature representations that are most suitable for two modalities of images, aiming to reduce the differences between modalities. Wu et al. [29] defined the cross-modal person ReID in 2017 and divided models into single-stream, dual-stream, and asymmetric fully connected layers. Ye et al. [18,30] employed the dual-stream network to separately extract shared information from the two modalities. They achieved feature fusion through feature mapping to the same space during the feature embedding process. Building on this foundation, Liu et al. [13] merged intermediate layer features to enhance cross-modal information integration. Zhang et al. [31] introduced a dual-path modality framework for feature extraction. This approach preserved the dual-path spatial network and its structure in the common space while focusing on the co-variance of input cross-modal images to deeply explore feature correlations. Additionally, the methods mentioned above, attention mechanisms have also been applied to assist in the process of feature learning. For example, Wang et al. [32] proposed an Attention-based Dual Stream Modality-aware method (ADSM) to effectively learn the same-modality inter-class differences. However, although feature representation learning has made certain progress, its performance on large-scale datasets has not yet reached a high level.
Different from feature learning, metric learning not only mines shared information through various network frameworks, but also requires the design of an effective metric learning method or distance metric loss function. These methods aim to constrain the training process of the model and narrow the distances for single- and cross-modal cases. Ultimately, more effective cross-modal ReID models are formed for accurate detection and identification. Wu et al. [24] proposed a focus-aware similarity preservation loss to guide the learning of modality-similar features. Zhao et al. [15] introduced a Hard Quintuplet Loss, composed of global triplet loss and difficult cross-modal triplet functions. Similarly, Liu et al. [13] designed a Dual-Modal Triplet Loss. All of these approaches contributed to improving the accuracy of VI-ReID to a certain extent.
The concept of center loss has been widely employed in VI-ReID. For instance, Zhu et al. [16] introduced the Asymmetric Center Loss, which reduced cross-modal differences between classes and avoided excessive computation. Additionally, more recent improvements were based on heterogeneous centers and difficult triplet losses. Liu et al. [17] proposed the Heterogeneous Center Triplet Loss and similar approaches. These methods optimized learning through the design of loss functions or network models. However, most of the above methods employed simple slices and weightless constraints on local features, and there remains limited focus on addressing the loss due to multi-granularity information differences.
Kniaz et al. introduce a new direction in VI-ReID through image generation based on Generative Adversarial Networks (GANs) [33]. They transformed visible light images into infrared images to supplement the dataset. In [34], the authors utilized the adversarial training concept of GANs, proposing a cross-generative adversarial network based on adversarial training to eliminate variations in cross-modal images. To bridge the cross-modal gap, in [35], Wang et al. presented a relief learning framework based on bidirectional cycle generators. Choi et al. [36] proposed the Hierarchical Intra-Cross-Modal Disentanglement (Hi-CMD) method, which automatically extracted key identification information from both infrared and visible modalities to reduce the impact of cross-modal information disparities. Wei et al. [37] proposed the Flexible Body Partition (FBP) model-based adversarial learning method (FBP-AL) to focus on designing modality classifiers to automatically distinguish part representations for obtaining more effective modality-sharable features. This also included the recent work by Zhong et al. [23], who proposed the Grayscale Enhancement Colorization Network (GEC-Net) for VI-ReID. It assisted in learning cross-modal image information by generating intermediate grayscale images.
However, although the above methods have improved recognition efficiency and accuracy, image-based generation methods still suffer from uncertainties in performance due to the disregard of critical details (such as color information) and limitations in model access.
2.4 Multi-Granularity Shared Feature Mining
The current methods of feature learning ignore the enormous potential inherent in multi-granularity information. Significant achievements have been made in the field of VI-ReID to realize multi-granularity feature learning by simultaneously focusing on local and global features. As illustrated in the GLMC network by [28], it has been attempted to extract multi-granularity features to reconcile global and local parts by focusing on both coarse and detailed information simultaneously. The study by Tang et al. [8] summarized the general approach to local feature extraction, which was to partition the output feature of the network on a horizontal scale. This research suggested that the method is too coarse. It not only overemphasized the noise information but also destroyed the scale structure of the normal pedestrian.
While existing methods have used the MGN network from person VV-ReID to achieve multi-granularity feature learning, they have not considered the key issue in VI-ReID, which is addressing the substantial inter-class differences. Our designed network takes this into account and utilizes an innovative disentanglement approach to enable the fusion module to learn multi-granularity features more efficiently and accurately. It not only improves the application of multi-granularity feature learning in VI-ReID but also provides a new direction for designing feature networks with multi-granularity and multi-scale learning capabilities.
The architecture designed for VI-ReID, termed Augmented Deep Multi-granularity Pose-aware Feature Fusion Network (ADMPFF-Net), is illustrated in Fig. 1. Inspired by HcTri [17], the main architectural framework of this network is based on the classical dual-stream architecture. Therefore, the method proposed in HcTri [17] can be regarded as the baseline of VI-ReID. The backbone of ADMPFF-Net is ResNet-50, which is pre-trained on the ImageNet dataset. ADMPFF-Net consists of four main modules, namely (1) dual-stream specific and shared feature extraction module, (2) multi-granularity pose-aware feature fusion module, (3) local information enhancement module, and (4) multi-granularity joint loss module. The integration of these modules aims to achieve discriminative and adaptive multi-granularity feature representation learning in the VI-ReID task, thus enhancing the accuracy of VI-ReID. The following subsections provide detailed descriptions of these key techniques.
Figure 1: Framework of the proposed ADMPFF-Net, which includes the feature separation module represented by light blue, feature fusion represented by yellow, the disentanglement part represented by green and dark blue, and the augmented part represented by orange. Rectangles of the same color represent feature stripes within the same branch. The arrows point to the losses applied to the feature stripes at the end
3.1 Dual-Stream Network for Specific and Shared Feature Learning
The dual-stream feature extraction network is widely used to extract cross-modal information [30]. It can be divided into two parts: feature extraction and fusion. The feature extractor is responsible for obtaining shallow-level specific features from the two heterogeneous inputs by using two separate branches. The feature fusion part then projects these specific features into a common space to uncover more effective modal-shared features. However, existing research just employs feature fusion through sharing the last convolution layers or fully connected layers, thus resulting in shallow and less efficient feature fusion.
To address the above-mentioned issue, during the specific design process of the network, stages 0 to i of the model serve as feature extraction layers to process the input images. Therefore, the shallow modality-specific features from the two different modalities can be obtained. The visible light and infrared feature extraction branches are represented as functions
In Eq. (1), the parameters
3.2 Multiple-Granularity Pose-Aware Feature Fusion
When both coarse- and fine-grained information is deeply integrated into the model, there is a problem of model oscillation that tends to be magnified. This is probably due to the large differences between the information content of the different granularity features. Moreover, existing research typically involves the horizontal proportional segmentation of network output features. Nevertheless, this method may overly focus on the noisy information, thereby destroying the body structure in normal proportions of the pedestrian. Therefore, ADMPFF-Net introduces Multiple-granularity Pose-aware Feature Fusion (MPFF) module for VI-ReID. Instead of employing the global feature, MPFF adopts a strategy that connects segmented local features to form a complete coarse-grained output. It consists of two main components, as shown in Fig. 1 below, namely the multi-granularity feature disentanglement strategy (mGFD) and the posture information segmentation strategy (pIS). These strategies correspond to two multi-granularity feature extraction branches and one local feature enhancement branch. Each branch not only strips local features from the image but also connects all of them to form a global representation. The local feature enhancement branch segments the feature using the corresponding method and selects the high-performance representation as the augmented part of the local feature. For all feature strips, a
3.2.1 Multi-Granularity Feature Disentanglement Strategy
We explain mGFD for Strategy 1 shown in Table 1. In table, the Disentanglement part of the first stage denotes the stage after this layer as the unshared parameter module copied for extracting multi-granularity information; stage0–1 denotes the layer of stage0 to stage1 as the stage contained in this module. For scheme 1, we set the stage0 as a dual-stream specific feature extraction module for cross-modal images; stages1–3 are utilized to obtain the shared feature with feature fusion, and the final stage4 is copied into three independent subspaces for disentangling and learning different granularity information. Two of these subspaces serve as multi-granularity modules, while one functions as the local feature enhancement module. Schemes 2, 3, and 4 follow a similar pattern, each with its respective purpose.
The introduction of mGFD reduces the disruption of structural information by different detailed data. We denote the multi-granularity feature module as
The details about the subsequent processing of the output features by the network are illustrated in Fig. 2. It is mainly divided into 3 major steps. (1) The max-pooling layer is employed to reduce the dimensionality of the original features; (2) The
Figure 2: Implementation details of multi-granularity feature disentanglement strategy
In order to better construct a test feature that comprehensively reflects the overall network learning status and at the same time ensures that the constructed feature is more stable and conforms to the correct human structure, the construction process begins by concatenating the complete pedestrian features
It can be observed that mGFD is different from conventional strategies for global feature learning. Existing strategies are developed from VV-ReID and utilize independent branches for global feature extraction. The mGFD module selectively segments local features into sub-features, which are then fused to generate global features. Specifically, it employs two different branches to extract three and six sub-features, respectively. After subdivision, these features are recombined to form a complete global feature, each of which is subject to an independent loss constraint. Additional details about these features can be seen in Table 2. This strategy on the one hand enables comprehensive multi-granularity information extraction, and, on the other hand, enhances the stability of the model parameters.
3.2.2 Posture Information Segmentation Strategy
The most common method for extracting local features is to divide the feature map into equal parts. The parameter
Figure 3: The difference between pose-aware segmentation and equal segmentation is demonstrated in (a) and (b). Pedestrians in (a) and (b) are from visible and infrared modalities, respectively. The red and blue boxes represent the torso and lower body of the original human structure, respectively
By analyzing the pedestrian images in the VI-ReID dataset, it can be observed that when p = 3, the typical proportions of a pedestrian’s head, upper body, and lower body in the images are approximately 1:2:3. Based on it, we adopt a novel feature segmentation strategy named pIS for local feature extraction. Its main purpose is to partition the output features into different stripes in the ratio of 1:2:3, which corresponds to the normal proportions of the human head, torso, and lower body. Compared with other non-equal segmentation strategies, such as the method proposed in [8], which combines fused features and gait energy images (GEI), pIS not only accurately captures complex body details, but also avoids introducing excessive parameters. Consequently, it can be applied more effortlessly and efficiently in multi-scale feature learning tasks.
3.3 Local Information Enhancement Module
In order to emphasize the role of important information, we designed a LIE module by adding a detached branch for VI-ReID. Obviously, there is a great difference in the distinctiveness and saliency of local features at different locations. Therefore, to emphasize the highly expressive local feature, we introduced a LIE module for the cross-modality task. In the detail of the model design, an independent branch for local feature enhancement is proposed following the process of the mGFD strategy. This enables repetitive reinforcement learning of the specific feature.
Based on observations from datasets and extensive exploration of local features, we analyze the representational ability of local parts of pedestrians, and the results are presented in Fig. 4. It uses the green boxes to highlight regions with strong representational capabilities, while the red boxes represent structures that are less discriminative for different pedestrians. When the local features are divided into three parts, the features of the head and lower body are represented significantly higher than the torso. Similarly, the local features perform relatively well when divided into six parts, except for the region of the thighs and abdomen. Therefore, it is important to reinforce the critical region from the shallow layer of the network using a separate branch to improve the model discrimination. we analyze the representational ability of local parts of pedestrians, and the results are presented in Fig. 4.
Figure 4: The red boxes are labeled as lower-resolution structures and the green boxes are labeled as higher-resolution structures
The attention mechanism adjusts the weights by automatically calculating the feature weights through training. However, it tends to determine the importance of deep-level features. Therefore, attention mechanism may ignore the important role played by mid-level semantic information in judging whether it is the same pedestrian or not. Unlike the attention mechanism, LIE is based on the analysis of pedestrian images and strengthens from the mid-level features, which is more consistent with the description of human structure. In the paper’s experiments, we show the performance difference between using the attention mechanism and the LIE module.
3.4 Multi-Granularity Joint Loss
In order to further enhance the similarity between the same pedestrian, a novel metric loss named multi-granularity joint (MGJ) loss is proposed for VI-ReID. We use ID loss and heterogeneous center triplet loss (
where
However, it is difficult to just rely on the ID loss to enhance the discrimination of the network in handling homogeneous and heterogeneous information simultaneously. For this reason, the triplet loss is widely used in the Re-ID task, which was originally introduced in FaceNet [38]. It is subsequently improved through various methods, such as the mining hard triplets [39].
where
where
Under the single granularity condition, these losses jointly lead to good performance. However, during multi-granularity feature learning, the information content of coarse-and fine-grained features is different due to varying slice sizes. In turn, this may cause the network to fall into oscillations. In order to enhance the stability of learning and enable the model to converge faster, we introduce the multi-granularity joint loss for VI-ReID. The underlying principle of the proposed loss is also similar to the loss used in mainstream multi-granularity methods. By jointly constraining the fine-grained local and coarse-grained global features with ID loss and triplet loss, respectively. The details are as follows:
where
The two weight parameters
By conducting comparison and ablation experiments, this section mainly evaluates the performance improvements of the proposed method on two publicly available datasets, including RegDB and SYSU-MM01. It also describes the detailed experimental settings and experimental criteria.
SYSU-MM01 serves as a large-scale cross-modal pedestrian dataset. It comprises data from 6 cameras, including 4 visible cameras and 2 infrared cameras. The training set contains 395 pedestrians, including 22,258 visible light images and 11,909 infrared images. And the testing set consists of an additional 96 individuals, including 3,803 infrared images for queries and 301 randomly selected visible images for the gallery set. Additionally, this dataset is positioned in indoor and outdoor settings. These two search modes represent distinct challenges. The evaluation involves 10 gallery set selection trials and reports the average retrieval performance. Detailed descriptions of the evaluation protocol can be found in [17].
RegDB is another extensively used dataset. It is established by a dual-camera system (one visible camera and one thermal camera) and contains 412 individual identities. Each person has 10 visible images and 10 thermal images. Following the evaluation protocols used in [17], the dataset is randomly divided into training and testing subsets. During testing, images from one modality (thermal images) are utilized as the gallery set, while images from the other modality (visible images) serve as the query set. This process is repeated 10 times for result stability.
During the training process, we adopt Cumulative Matching Characteristics (CMC), mean Average Precision (mAP), and mean Inverse Negative Penalty (mINP) [2] as evaluation protocols for the effectiveness of the model. It is worth noting that before testing, the features of all IDs are first subjected to L2 Norm to ensure the consistency and comparability of the data. These evaluation protocols validate the mode’s effectiveness and provide powerful support and reference for this study.
The proposed network is implemented on the NVIDIA RTX4090 GPU with PyTorch. Based on previous research and experimentation, the network selected a pretrained ResNet50 with ImageNet weights as the backbone network. In order to capture more detailed information, we adjusted the step size of the final convolution block during training from 2 to 1. This adjustment enlarges the feature map by 4 times. During the training process, the size of the input image is set to
For optimizer selection, we employ the stochastic gradient descent (SGD) optimizer. The momentum is set to 0.9, and the initial learning rate is set to 0.1. Furthermore, the experiment utilizes a warm-up learning rate strategy, which helps to speed up the convergence of the network and achieve superior performance. The specific learning rate settings are presented below:
Other hyper-parameters are outlined in Table 3. Moreover, the total number of training epochs is 80.
In order to demonstrate the effectiveness of the method proposed in this paper, we perform ablation experiments on public datasets. The experiments are employed to evaluate the effectiveness of different components in ADMPFF-Net, including MPFF, LIE, and multi-granularity joint loss.
4.2.1 Effectiveness of MPFF Module
MPFF consists of two main components: Multi-granularity Feature Disentanglement Strategy (mGFD) and Posture Information Segmentation Strategy (pIS). In the following, specific experiments verify the effectiveness of the two strategies in multi-granularity feature learning, respectively.
Effectiveness of mGFD Strategy: The central part of the mGFD strategy is the design of the disentanglement module. The key to effectively extracting deep multi-granularity features lies in the appropriate stage selection of the disentanglement module. As shown in Table 4, when the shared module is configured as stages2–4 without the disentanglement module, the performance on SYSU-MM01 is significantly lower than the others. This indicates that mGFD effectively promotes feature learning. Furthermore, the experimental results show that when the fusion modules become too large, the accuracy of the model in handling multi-granularity features may decrease, leading to a decline in performance. This aligns with the results where the best performance is achieved when stages 1–2 are set as the shared module (stages 3–4 as the disentanglement module). Compared to other settings of the disentanglement module, SYSU-MM01 shows a significant improvement of 3% to 4%. This finding emphasizes the importance of reasonable control over the disentanglement module in multi-granularity feature fusion and provides further experimental validation for the approach proposed in this paper.
Effectiveness of pIS Strategy: The pIS strategy offers a new approach for extracting more discriminative local features. When compared to traditional equal segmentation methods, even finer divisions such as 6 parts or 9 parts, pIS proposed in this study can explore human body structural information and perform feature segmentation based on more precise and reasonable proportions. As depicted in Table 5, pIS can be observed as an improvement of nearly 3% in rank-1 on the SYSU-MM01 dataset. Additionally, achieves even more significant enhancements in the mAP and mINP accuracy, with improvements exceeding 9%. Hence, in comparison to mainstream strategies, the pIS segmentation strategy exhibits significant advantages in terms of computational cost and feature segmentation efficiency.
4.2.2 Effectiveness of LIE Module
To explore the effectiveness and optimal performance of the LIE Module, the following experiments were conducted, focusing on both network branch configuration and module feature selection.
Effectiveness of Network Branch Configuration: ADMPFF-Net focuses on emphasizing features with better representation. Mainstream networks often employ separate global branch (Global part)) to fully extract coarse-grained information. As shown in Fig. 5 below, such methods achieve results of 63.32% for rank-1, 61.47% for mAP, and 48.04% for mINP accuracy. In contrast, this paper proposes using the LIE branch as an alternative. On the one hand, the attention mechanism attempts to focus on features with higher expressiveness through the learning of feature weights but ignores the mid-level semantic information. On the other hand, our proposed LIE module starts from the mid-level features and enhances the attention to the more structurally superior features. To verify the effectiveness of the LIE branch, the study conducts a series of experiments to compare with different cases. The cases use the attention mechanism branch (Attention), the global feature branch (Global part) and non-branch (Non). As shown in the results in Fig. 5 below, the performance of the LIE branch configuration significantly surpasses other network branch configuration methods on rank-1, mAP and mINP accuracy.
Figure 5: Results on SYSU-MM01, the cases with the Attention, Global part and Non are employed to compare with LIE
Effectiveness of Feature Selection in LIE: The feature selection experiments for the LIE module involve two major steps: identifying specific high-performance structures and determining the corresponding feature sizes. Firstly, as indicated in Fig. 6a, the feature of the torso has relatively low discrimination. In comparison, the head and leg features exhibit better performance and reflect human body information more effectively. Secondly, by applying different segmentation sizes to the optimally performing leg features, the optimal feature map learning size is determined. Combining the experimental results in Fig. 6b, it is observed that on the SYSU-MM01 dataset, the leg feature size of
Figure 6: (a) Depicts the effectiveness of different structural features extracted from the human head, upper body, and lower body; (b) presents performance variations under different sizes that explore the optimal structure
4.2.3 Effectiveness of the MGJ Loss
In this subsection, related experiments primarily validate the effectiveness of our proposed Multi-granularity Joint Loss from two perspectives. On the one hand, different from other multi-granularity feature learning methods, the coarse-grained part of ADMPFF-Net is generated through recombining and connecting local features. It is individually constrained by a specialized loss. Therefore, it is essential to demonstrate the efficacy of this individual loss in constraining coarse-grained information. On the other hand, it is important to establish the superiority of the proposed
Effectiveness of Coarse-Grained Loss: This experiment evaluates the efficiency of multi-granularity feature extraction compared to single local or global features under the same network architecture and parameter settings. In the specific loss construction process, we primarily utilize individual losses to constrain the global features formed by connected local features, achieving the Coarse-grained Loss. The result in Table 6 presents that just using local features outperforms employing global features due to the effective enhancement from LIE. Moreover, the most optimal results are achieved by individually constraining the coarse and fine-grained losses, showcasing improvements of over 4% on rank-1, mAP and mINP compared to others. This robustly demonstrates the superiority of learning multi-granularity information.
Effectiveness of
4.3 Comparison with State-of-the-Art Methods
This section primarily focuses on a comprehensive comparison between our proposed ADMPFF-Net and several SOTA VI-ReID methods. A detailed comparative analysis on the RegDB and SYSU-MM01 datasets is presented in Tables 8 and 9. The experimental results demonstrate that the proposed ADMPFF-Net can significantly improve the recognition and learning of cross-modal pedestrian images.
The experiments on the RegDB dataset are shown in Table 8, where the proposed method achieves the best performance in both query modes. The performance in Visible to Thermal mode is even optimized to rank1/mAP/mINP with 93.54%/85.02%/70.82%, which is an effective improvement over other methods. For example, compared to GECNet [23], ADMPFF-Net demonstrates performance advantages of 11.21% in rank-1 and 6.57% in mAP. When compared to the baseline HcTri [17], ADMPFF-Net exhibits performance improvements of 2.49% in rank-1, 1.74% in mAP, and 1.98% in mINP. This simultaneously demonstrates the effectiveness of the multi-granularity loss used in this paper in guiding multi-granularity learning. Even when compared to the latest methods such as FBP-Al [38], the improvements in various metrics are also quite significant. These studies validate the effectiveness of the multi-granularity feature learning framework used in this research.
On the SYSU-MM01 dataset, we compare various methods with ADMPFF-Net, and the specific results are presented in Table 9. From the table, it can be observed that the proposed method achieves notable improvements over the baseline method HcTri [17]. The rank-1, mAP, and mINP accuracy can be improved from 61.68%, 57.51%, and 39.54% to 67.66%, 63.5%, and 49.70%, respectively. Additionally, in comparison with GLMC [28], a method with a larger parameter size but a similar focus on multi-scale information extraction, our proposed method achieved a 3.29% improvement in the rank-1 accuracy. Furthermore, when compared to the latest methods such as ADSM [34], ADMPFF-Net exhibits significant superiority, with improvements in relevant metrics exceeding 10%. What’s more, it substantially reduces the relative computational load and computation time. Therefore, ADMPFF-Net making it exceptionally outstanding for practical applications.
This study introduces ADMPFF-Net, a novel network designed to optimize the VI-ReID model for extracting discriminative and robust multi-granularity information about pedestrians. ADMPFF-Net improves upon the dual-stream feature learning network by introducing the MPFF module. This module incorporates the mGFD and pIS strategies, allowing the extraction of both coarse- and fine-grained information from pedestrian images in a cross-modal setting. These strategies greatly enhance the efficiency and accuracy of mining multi-granularity information, offering an innovative and effective approach to designing multi-granularity feature learning networks. In addition, ADMPFF-Net can be applied as a novel baseline for recognition tasks in scenarios of street, station and campus.
Furthermore, the proposed method integrates the LIE module, which adds separate branches behind the middle layer of the network. It enhances the role of high-performance features and promotes stability in the overall feature-learning process.
To facilitate effective feature learning in VI-ReID, the proposed multi-granularity joint loss is employed to supervise model training. This loss function focuses on extracting more discriminative multi-granularity features. Through extensive experiments conducted on two prominent VI-ReID datasets, the results demonstrate that the proposed method effectively constructs pedestrian feature representations, leading to improved recognition accuracy.
Acknowledgement: We want to thank the team at Sun Yat-sen University for creating the SYSU dataset and the Hong Kong Polytechnic University for providing the RegDB dataset. This has laid a critical foundation for our research and experiments.
Funding Statement: This work is supported in part by the National Natural Science Foundation of China under Grant 62177029 and 62307025, in part by the Startup Foundation for Introducing Talent of Nanjing University of Posts and Communications under Grant NY221041, and in part by the General Project of The Natural Science Foundation of Jiangsu Higher Education Institution of China 22KJB520025 and 23KJD580.
Author Contributions: The authors confirm contribution to the paper as follows: study conception and design: Z. Shi and W. Song; data collection: F. Liu and J. Shan; analysis and interpretation of results: Z. Shi and W. Song; draft manuscript preparation: W. Song, Z. Shi. All authors reviewed the results and approved the final version of the manuscript.
Availability of Data and Materials: The additional datasets generated during and analyzed during the current study are available from the corresponding author upon reasonable request.
Conflicts of Interest: The authors declare that they have no conflicts of interest to report regarding the present study.
References
1. L. Zheng, Y. Yang and A. G. Hauptmann, “Person re-identification: Past, present and future,” arXiv:1610.02984, 2016. [Google Scholar]
2. M. Ye, J. Shen, G. Lin, T. Xiang, L. Shao et al., “Deep learning for person re-identification: A survey and outlook,” IEEE Transactions on Pattern Analysis and Machine Intelligence, vol. 44, no. 6, pp. 2872–2893, 2022. [Google Scholar] [PubMed]
3. N. Huang, J. Liu, Y. Miao, Q. Zhang and J. Han, “Deep learning for visible-infrared cross-modality person re-identification: A comprehensive review information,” Information Fusion, vol. 91, pp. 396–411, 2023. [Google Scholar]
4. S. Ki Kim, T. Kim and K. Kim, “Analysis of teaching and learning environment for data science and AI education (Focused on 2022 revised curriculum),” in 2023 Int. Conf. on Artificial Intelligence in Information and Communication, Bali, Indonesia, pp. 788–790, 2023. [Google Scholar]
5. S. U. Khan, I. U. Haq, N. Khan, A. Ullah, K. Muhammad et al., “Efficient person re-identification for IoT-assisted cyber-physical systems,” IEEE Internet of Things Journal, vol. 10, no. 21, pp. 18695–18707, 2023. [Google Scholar]
6. Y. Liu, J. Yan and W. Ouyang, “Quality aware network for set to set recognition,” in 2017 IEEE Conf. on Computer Vision and Pattern Recognition, Hawaii, USA, pp. 4694–4703, 2017. [Google Scholar]
7. J. Dai, P. Zhang, D. Wang, H. Lu and H. Wang, “Video person re-identification by temporal residual learning,” IEEE Transactions on Image Processing, vol. 28, no. 3, pp. 1366–1377, 2019. [Google Scholar]
8. X. Tang, X. Sun, Z. Wang, P. Yu and N. Cao, “Research on the pedestrian re-identification method based on local features and gait energy images,” Computers, Materials & Continua, vol. 64, no. 2, pp. 1185–1198, 2020. [Google Scholar]
9. D. Cheng, Y. Gong, S. Zhou, J. Wang and N. Zheng, “Person re-identification by multi-channel parts-based CNN with improved triplet loss function,” in 2016 IEEE Conf. on Computer Vision and Pattern Recognition, Las Vegas, LV, USA, pp. 1335–1344, 2016. [Google Scholar]
10. H. Yao, S. Zhang, R. Hong, Y. Zhang, C. Xu et al., “Deep representation learning with part loss for person re-identification,” IEEE Transactions on Image Processing, vol. 28, no. 6, pp. 2860–2871, 2019. [Google Scholar]
11. H. Liu, Y. Chai, X. Tan, D. Li and X. Zhou, “Strong but simple baseline with dual-granularity triplet loss for visible-thermal person re-identification,” IEEE Signal Processing Letters, vol. 28, pp. 653–657, 2021. [Google Scholar]
12. Y. Hao, N. Wang, X. Gao, J. Li and X. Wang, “Dual-alignment feature embedding for cross-modality person re-identification,” in 2019 ACM Int. Conf. on Multimedia, New York, NY, USA, pp. 57–65, 2019. [Google Scholar]
13. H. Liu, J. Cheng, W. Wang, Y. Su and H. Bai, “Enhancing the discriminative feature learning for visible-thermal cross-modality person re-identification,” Neurocomputing, vol. 398, pp. 11–19, 2020. [Google Scholar]
14. Y. Cheng, G. Xiao, X. Tang, W. Ma and X. Gou, “Two-phase feature fusion network for visible-infrared person re-identification,” in 2021 IEEE Int. Conf. on Image Processing, Anchorage, AK, USA, pp. 1149–1153, 2021. [Google Scholar]
15. Y. B. Zhao, J. W. Lin, Q. Xuan and X. Xi, “HPILN: A feature learning frame-work for cross-modality person re-identification,” IET Image Processing, vol. 13, pp. 2897–2904, 2019. [Google Scholar]
16. Y. Zhu, Z. Yang, L. Wang, S. Zhao, X. Hu et al., “Hetero-center loss for cross-modality person re-identification,” Neurocomputing, vol. 386, pp. 97–109, 2020. [Google Scholar]
17. H. Liu, X. Tan and X. Zhou, “Parameter sharing exploration and hetero-center triplet loss for visible-thermal person re-identification,” IEEE Transactions on Multimedia, vol. 23, pp. 4414–4425, 2021. [Google Scholar]
18. M. Ye, X. Lan, Z. Wang and P. C. Yuen, “Bi-directional center-constrained top-ranking for visible thermal person re-identification,” IEEE Transactions on Information Forensics and Security, vol. 15, pp. 407–419, 2020. [Google Scholar]
19. H. Ye, H. Liu, F. Meng and X. Li, “Bi-directional exponential angular triplet loss for RGB-infrared person re-identification,” IEEE Transactions on Image Processing, vol. 30, pp. 1583–1595, 2021. [Google Scholar] [PubMed]
20. D. Li, X. Wei, X. Hong and Y. Gong, “Infrared-visible cross-modal person re-identification with an x modality,” in Proc. of the AAAI Conf. on Artificial Intelligence, vol. 34, no. 4, pp. 4610–4617, 2020. [Google Scholar]
21. Y. Zhang, Y. Yan, Y. Lu and H. Wang, “Towards a unified middle modality learning for visible-infrared person re-identification,” in 2021 ACM Int. Conf. on Multimedia, New York, NY, USA, pp. 788–796, 2021. [Google Scholar]
22. M. Ye, W. Ruan, B. Du and M. Z. Shou, “Channel augmented joint learning for visible-infrared recognition,” in 2021 IEEE/CVF Int. Conf. on Computer Vision, Montreal, QC, Canada, pp. 13547–13556, 2021. [Google Scholar]
23. X. Zhong, T. Lu, W. Huang, M. Ye, X. Jia et al., “Grayscale enhancement colorization network for visible-infrared person re-identification,” IEEE Transactions on Circuits and Systems for Video Technology, vol. 32, no. 3, pp. 1418–1430, 2022. [Google Scholar]
24. A. Wu, W. S. Zheng, S. Gong and J. Lai, “RGB-IR person re-identification by cross-modality similarity preservation,” 2020 International Journal of Computer Vision, vol. 128, pp. 1765–1785, 2020. [Google Scholar]
25. M. Ye, J. Shen, D. J. Crandall, L. Shao and J. Luo, “Dynamic dual-attentive aggregation learning for visible-infrared person re-identification,” in 2020 European Conf. on Computer Vision, Cham, UK, Springer, vol. 12362, 2020. [Google Scholar]
26. J. Zhao, H. Wang, Y. Zhou, R. Yao, S. Chen et al., “Spatial-channel enhanced transformer for visible-infrared person re-identification,” IEEE Transactions on Multimedia, vol. 25, pp. 3668–3680, 2023. [Google Scholar]
27. G. Wang, Y. Yuan, X. Chen, J. Li and X. Zhou, “Learning discriminative features with multiple granularities for person re-identification,” in 2021 ACM Int. Conf. on Multimedia, Seoul, Korea, pp. 274–282, 2018. [Google Scholar]
28. L. Zhang, G. Du, F. Liu, H. Tu and X. Shu, “Global-local multiple granularity learning for cross-modality visible-infrared person reidentification,” IEEE Transactions on Neural Networks and Learning Systems, pp. 1–11, 2021. [Google Scholar]
29. A. Wu, W. S. Zheng, H. X. Yu, S. Gong and J. Lai, “RGB-infrared cross-modality person re-identification,” in 2017 IEEE Int. Conf. on Computer Vision, Venice, Italy, pp. 5390–5399, 2017. [Google Scholar]
30. M. Ye, Z. Wang, X. Lan and P. C. Yuen, “Visible thermal person re-identification via dual-constrained top-ranking,” in 2018 Int. Joint Conf. on Artificial Intelligence, Stockholm, Sweden, pp. 1092–1099, 2018. [Google Scholar]
31. S. Zhang, Y. Yang, P. Wang, G. Liang, X. Zhang et al., “Attend to the difference: Cross-modality person re-identification via contrastive correlation,” IEEE Transactions on Image Processing, vol. 30, pp. 8861–8872, 2021. [Google Scholar] [PubMed]
32. H. Wang and W. Wu, “Attention based dual stream modality-aware model for visible-infrared person re-identification,” in 2023 Int. Conf. on Computer Supported Cooperative Work in Design, Rio de Janeiro, Brazil, pp. 897–902, 2023. [Google Scholar]
33. V. Kniaz, V. Knyaz, J. Hladůvka, W. Kropatsch and V. Mizginov, “ThermalGAN: Multimodal color-to-thermal image translation for person re-identification in multispectral dataset,” in 2018 European Conf. on Computer Vision, Munich, Germany, pp. 606–624, 2018. [Google Scholar]
34. P. Dai, R. Ji, H. Wang, Q. Wu and Y. Huang, “Cross-modality person re-identification with generative adversarial training,” in 2018 Int. Joint Conf. on Artificial Intelligence, Stockholm, Sweden, pp. 677–683, 2018. [Google Scholar]
35. Z. Wang, Z. Wang, Y. Zheng, Y. Y. Chuang and S. Satoh, “Learning to reduce dual-level discrepancy for infrared-visible person re-identification,” in 2019 IEEE/CVF Conf. on Computer Vision and Pattern Recognition, Long Beach, CA, USA, 2019. [Google Scholar]
36. S. Choi, S. Lee, Y. Kim, T. Kim and C. Kim, “Hi-CMD: Hierarchical cross-modality disentanglement for visible-infrared person re-identification,” in 2020 IEEE/CVF Conf. on Computer Vision and Pattern Recognition, Seattle, WA, USA, pp. 10254–10263, 2020. [Google Scholar]
37. Z. Wei, X. Yang, N. Wang and X. Gao, “Flexible body partition-based adversarial learning for visible infrared person re-identification,” IEEE Transactions on Neural Networks and Learning Systems, vol. 33, no. 9, pp. 4676–4687, 2022. [Google Scholar] [PubMed]
38. F. Schroff, D. Kalenichenko and J. Philbin, “FaceNet: A unified embedding for face recognition and clustering,” in 2015 IEEE Conf. on Computer Vision and Pattern Recognition, Boston, MA, USA, pp. 815–823, 2015. [Google Scholar]
39. A. Hermans, L. Beyer and B. Leibe, “In defense of the triplet loss for person re-identification,” arXiv:1703.07737, 2017. [Google Scholar]
Cite This Article
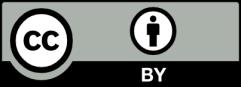