Open Access
ARTICLE
Design of a Lightweight Compressed Video Stream-Based Patient Activity Monitoring System
1 Department of Computer Science & Applications, Maharshi Dayanand University, Rohtak, 124001, India
2 University Institute of Technology, Rajiv Gandhi Proudyogiki Vishwavidyalaya, Bhopal, 462033, India
3 Department of Computer Science, College of Computing, Khon Kaen University, Khon Kaen, 40002, Thailand
4 Department of Computer Science, College of Computer and Information Sciences, Majmaah University, Al Majmaah, 11952, Kingdom of Saudi Arabia
5 Department of Computer Engineering, College of Computer and Information Sciences, Majmaah University, Al Majmaah, 11952, Kingdom of Saudi Arabia
* Corresponding Authors: Preeti Gulia. Email: ; Nasib Singh Gill. Email:
(This article belongs to the Special Issue: Deep Learning based Object Detection and Tracking in Videos)
Computers, Materials & Continua 2024, 78(1), 1253-1274. https://doi.org/10.32604/cmc.2023.042869
Received 15 June 2023; Accepted 25 September 2023; Issue published 30 January 2024
Abstract
Inpatient falls from beds in hospitals are a common problem. Such falls may result in severe injuries. This problem can be addressed by continuous monitoring of patients using cameras. Recent advancements in deep learning-based video analytics have made this task of fall detection more effective and efficient. Along with fall detection, monitoring of different activities of the patients is also of significant concern to assess the improvement in their health. High computation-intensive models are required to monitor every action of the patient precisely. This requirement limits the applicability of such networks. Hence, to keep the model lightweight, the already designed fall detection networks can be extended to monitor the general activities of the patients along with the fall detection. Motivated by the same notion, we propose a novel, lightweight, and efficient patient activity monitoring system that broadly classifies the patients’ activities into fall, activity, and rest classes based on their poses. The whole network comprises three sub-networks, namely a Convolutional Neural Networks (CNN) based video compression network, a Lightweight Pose Network (LPN) and a Residual Network (ResNet) Mixer block-based activity recognition network. The compression network compresses the video streams using deep learning networks for efficient storage and retrieval; after that, LPN estimates human poses. Finally, the activity recognition network classifies the patients’ activities based on their poses. The proposed system shows an overall accuracy of approx. 99.7% over a standard dataset with 99.63% fall detection accuracy and efficiently monitors different events, which may help monitor the falls and improve the inpatients’ health.Keywords
Cite This Article
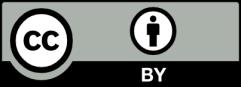