Open Access
ARTICLE
Deep Learning Approach for Hand Gesture Recognition: Applications in Deaf Communication and Healthcare
1 Department of Computer Engineering, College of Computer and Information Sciences, King Saud University, P.O.Box 51178, Riyadh, 11543, Kingdom of Saudi Arabia
2 Department of Computer Engineering, College of Computing and Informatics, University of Sharjah, Sharjah, 27272, United Arab Emirates
3 Laboratory Biomechanics and Bioengineering, University of Technology of Compiegne, Compiegne, 60200, France
4 Department of Computer Science and Engineering, Manipal University Jaipur, Jaipur, 303007, India
* Corresponding Author: Anubha Parashar. Email:
Computers, Materials & Continua 2024, 78(1), 127-144. https://doi.org/10.32604/cmc.2023.042886
Received 15 June 2023; Accepted 11 October 2023; Issue published 30 January 2024
Abstract
Hand gestures have been used as a significant mode of communication since the advent of human civilization. By facilitating human-computer interaction (HCI), hand gesture recognition (HGRoc) technology is crucial for seamless and error-free HCI. HGRoc technology is pivotal in healthcare and communication for the deaf community. Despite significant advancements in computer vision-based gesture recognition for language understanding, two considerable challenges persist in this field: (a) limited and common gestures are considered, (b) processing multiple channels of information across a network takes huge computational time during discriminative feature extraction. Therefore, a novel hand vision-based convolutional neural network (CNN) model named (HVCNNM) offers several benefits, notably enhanced accuracy, robustness to variations, real-time performance, reduced channels, and scalability. Additionally, these models can be optimized for real-time performance, learn from large amounts of data, and are scalable to handle complex recognition tasks for efficient human-computer interaction. The proposed model was evaluated on two challenging datasets, namely the Massey University Dataset (MUD) and the American Sign Language (ASL) Alphabet Dataset (ASLAD). On the MUD and ASLAD datasets, HVCNNM achieved a score of 99.23% and 99.00%, respectively. These results demonstrate the effectiveness of CNN as a promising HGRoc approach. The findings suggest that the proposed model have potential roles in applications such as sign language recognition, human-computer interaction, and robotics.Keywords
Cite This Article
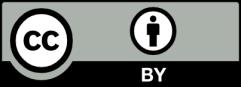