Open Access
ARTICLE
Lightweight Malicious Code Classification Method Based on Improved SqueezeNet
School of Computer and Control Engineering, Northeast Forestry University, Harbin, 150040, China
* Corresponding Author: Li Li. Email:
Computers, Materials & Continua 2024, 78(1), 551-567. https://doi.org/10.32604/cmc.2023.045512
Received 29 August 2023; Accepted 15 November 2023; Issue published 30 January 2024
Abstract
With the growth of the Internet, more and more business is being done online, for example, online offices, online education and so on. While this makes people’s lives more convenient, it also increases the risk of the network being attacked by malicious code. Therefore, it is important to identify malicious codes on computer systems efficiently. However, most of the existing malicious code detection methods have two problems: (1) The ability of the model to extract features is weak, resulting in poor model performance. (2) The large scale of model data leads to difficulties deploying on devices with limited resources. Therefore, this paper proposes a lightweight malicious code identification model Lightweight Malicious Code Classification Method Based on Improved SqueezeNet (LCMISNet). In this paper, the MFire lightweight feature extraction module is constructed by proposing a feature slicing module and a multi-size depthwise separable convolution module. The feature slicing module reduces the number of parameters by grouping features. The multi-size depthwise separable convolution module reduces the number of parameters and enhances the feature extraction capability by replacing the standard convolution with depthwise separable convolution with different convolution kernel sizes. In addition, this paper also proposes a feature splicing module to connect the MFire lightweight feature extraction module based on the feature reuse and constructs the lightweight model LCMISNet. The malicious code recognition accuracy of LCMISNet on the BIG 2015 dataset and the Malimg dataset reaches 98.90% and 99.58%, respectively. It proves that LCMISNet has a powerful malicious code recognition performance. In addition, compared with other network models, LCMISNet has better performance, and a lower number of parameters and computations.Keywords
Cite This Article
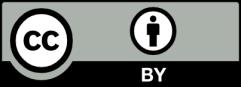