Open Access
ARTICLE
An Industrial Intrusion Detection Method Based on Hybrid Convolutional Neural Networks with Improved TCN
College of Computer Science and Technology, Xinjiang University, Xinjiang Uygur Autonomous Regoin, Urumqi, 830000, China
* Corresponding Author: Shengquan Liu. Email:
(This article belongs to the Special Issue: Cybersecurity for Cyber-attacks in Critical Applications in Industry)
Computers, Materials & Continua 2024, 78(1), 411-433. https://doi.org/10.32604/cmc.2023.046237
Received 24 September 2023; Accepted 15 November 2023; Issue published 30 January 2024
Abstract
Network intrusion detection systems (NIDS) based on deep learning have continued to make significant advances. However, the following challenges remain: on the one hand, simply applying only Temporal Convolutional Networks (TCNs) can lead to models that ignore the impact of network traffic features at different scales on the detection performance. On the other hand, some intrusion detection methods consider multi-scale information of traffic data, but considering only forward network traffic information can lead to deficiencies in capturing multi-scale temporal features. To address both of these issues, we propose a hybrid Convolutional Neural Network that supports a multi-output strategy (BONUS) for industrial internet intrusion detection. First, we create a multiscale Temporal Convolutional Network by stacking TCN of different scales to capture the multiscale information of network traffic. Meanwhile, we propose a bi-directional structure and dynamically set the weights to fuse the forward and backward contextual information of network traffic at each scale to enhance the model’s performance in capturing the multi-scale temporal features of network traffic. In addition, we introduce a gated network for each of the two branches in the proposed method to assist the model in learning the feature representation of each branch. Extensive experiments reveal the effectiveness of the proposed approach on two publicly available traffic intrusion detection datasets named UNSW-NB15 and NSL-KDD with F1 score of 85.03% and 99.31%, respectively, which also validates the effectiveness of enhancing the model’s ability to capture multi-scale temporal features of traffic data on detection performance.Keywords
Cite This Article
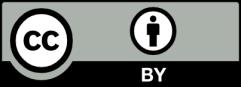