Open Access
ARTICLE
Detecting APT-Exploited Processes through Semantic Fusion and Interaction Prediction
1 School of Cyber Science and Engineering, Sichuan University, Chengdu, 610065, China
2 Key Laboratory of Data Protection and Intelligent Management (Sichuan University), Ministry of Education, Chengdu, 610065, China
3 Cyber Science Research Institute, Sichuan University, Chengdu, 610065, China
* Corresponding Authors: Shuhua Ruan. Email: ; Yonggang Luo. Email:
Computers, Materials & Continua 2024, 78(2), 1731-1754. https://doi.org/10.32604/cmc.2023.045739
Received 06 September 2023; Accepted 06 December 2023; Issue published 27 February 2024
Abstract
Considering the stealthiness and persistence of Advanced Persistent Threats (APTs), system audit logs are leveraged in recent studies to construct system entity interaction provenance graphs to unveil threats in a host. Rule-based provenance graph APT detection approaches require elaborate rules and cannot detect unknown attacks, and existing learning-based approaches are limited by the lack of available APT attack samples or generally only perform graph-level anomaly detection, which requires lots of manual efforts to locate attack entities. This paper proposes an APT-exploited process detection approach called ThreatSniffer, which constructs the benign provenance graph from attack-free audit logs, fits normal system entity interactions and then detects APT-exploited processes by predicting the rationality of entity interactions. Firstly, ThreatSniffer understands system entities in terms of their file paths, interaction sequences, and the number distribution of interaction types and uses the multi-head self-attention mechanism to fuse these semantics. Then, based on the insight that APT-exploited processes interact with system entities they should not invoke, ThreatSniffer performs negative sampling on the benign provenance graph to generate non-existent edges, thus characterizing irrational entity interactions without requiring APT attack samples. At last, it employs a heterogeneous graph neural network as the interaction prediction model to aggregate the contextual information of entity interactions, and locate processes exploited by attackers, thereby achieving fine-grained APT detection. Evaluation results demonstrate that anomaly-based detection enables ThreatSniffer to identify all attack activities. Compared to the node-level APT detection method APT-KGL, ThreatSniffer achieves a 6.1% precision improvement because of its comprehensive understanding of entity semantics.Keywords
Cite This Article
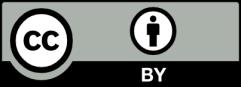