Open Access
ARTICLE
Advanced Optimized Anomaly Detection System for IoT Cyberattacks Using Artificial Intelligence
1 Department of Computer Science, Taibah University, Medina, 42315, Saudi Arabia
2 Department of Software Engineering, Kocaeli University, Kocaeli, 41001, Turkey
* Corresponding Author: Ali Hamid Farea. Email:
(This article belongs to the Special Issue: Advanced Data Mining Techniques: Security, Intelligent Systems and Applications)
Computers, Materials & Continua 2024, 78(2), 1525-1545. https://doi.org/10.32604/cmc.2023.045794
Received 07 September 2023; Accepted 30 November 2023; Issue published 27 February 2024
Abstract
While emerging technologies such as the Internet of Things (IoT) have many benefits, they also pose considerable security challenges that require innovative solutions, including those based on artificial intelligence (AI), given that these techniques are increasingly being used by malicious actors to compromise IoT systems. Although an ample body of research focusing on conventional AI methods exists, there is a paucity of studies related to advanced statistical and optimization approaches aimed at enhancing security measures. To contribute to this nascent research stream, a novel AI-driven security system denoted as “AI2AI” is presented in this work. AI2AI employs AI techniques to enhance the performance and optimize security mechanisms within the IoT framework. We also introduce the Genetic Algorithm Anomaly Detection and Prevention Deep Neural Networks (GAADPSDNN) system that can be implemented to effectively identify, detect, and prevent cyberattacks targeting IoT devices. Notably, this system demonstrates adaptability to both federated and centralized learning environments, accommodating a wide array of IoT devices. Our evaluation of the GAADPSDNN system using the recently complied WUSTL-IIoT and Edge-IIoT datasets underscores its efficacy. Achieving an impressive overall accuracy of 98.18% on the Edge-IIoT dataset, the GAADPSDNN outperforms the standard deep neural network (DNN) classifier with 94.11% accuracy. Furthermore, with the proposed enhancements, the accuracy of the unoptimized random forest classifier (80.89%) is improved to 93.51%, while the overall accuracy (98.18%) surpasses the results (93.91%, 94.67%, 94.94%, and 94.96%) achieved when alternative systems based on diverse optimization techniques and the same dataset are employed. The proposed optimization techniques increase the effectiveness of the anomaly detection system by efficiently achieving high accuracy and reducing the computational load on IoT devices through the adaptive selection of active features.Keywords
Cite This Article
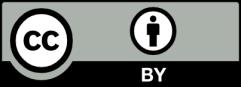