Open Access
ARTICLE
Learning Epipolar Line Window Attention for Stereo Image Super-Resolution Reconstruction
School of Information Engineering, Southwest University of Science and Technology, Mianyang, 621010, China
* Corresponding Author: Hongying Zhang. Email:
(This article belongs to the Special Issue: Development and Industrial Application of AI Technologies)
Computers, Materials & Continua 2024, 78(2), 2847-2864. https://doi.org/10.32604/cmc.2024.047093
Received 24 October 2023; Accepted 26 December 2023; Issue published 27 February 2024
Abstract
Transformer-based stereo image super-resolution reconstruction (Stereo SR) methods have significantly improved image quality. However, existing methods have deficiencies in paying attention to detailed features and do not consider the offset of pixels along the epipolar lines in complementary views when integrating stereo information. To address these challenges, this paper introduces a novel epipolar line window attention stereo image super-resolution network (EWASSR). For detail feature restoration, we design a feature extractor based on Transformer and convolutional neural network (CNN), which consists of (shifted) window-based self-attention ((S)W-MSA) and feature distillation and enhancement blocks (FDEB). This combination effectively solves the problem of global image perception and local feature attention and captures more discriminative high-frequency features of the image. Furthermore, to address the problem of offset of complementary pixels in stereo images, we propose an epipolar line window attention (EWA) mechanism, which divides windows along the epipolar direction to promote efficient matching of shifted pixels, even in pixel smooth areas. More accurate pixel matching can be achieved using adjacent pixels in the window as a reference. Extensive experiments demonstrate that our EWASSR can reconstruct more realistic detailed features. Comparative quantitative results show that in the experimental results of our EWASSR on the Middlebury and Flickr1024 data sets for 2 × SR, compared with the recent network, the Peak signal-to-noise ratio (PSNR) increased by 0.37 dB and 0.34 dB, respectively.Keywords
Cite This Article
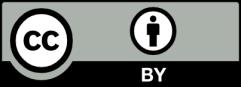