Open Access
ARTICLE
MSADCN: Multi-Scale Attentional Densely Connected Network for Automated Bone Age Assessment
1 College of Computer and Information Science, Chongqing Normal University, Chongqing, 401331, China
2 College of Mathematics and Statistics, Chongqing University, Chongqing, 401331, China
* Corresponding Author: Lei Yu. Email:
(This article belongs to the Special Issue: Deep Learning in Computer-Aided Diagnosis Based on Medical Image)
Computers, Materials & Continua 2024, 78(2), 2225-2243. https://doi.org/10.32604/cmc.2024.047641
Received 13 November 2023; Accepted 21 December 2023; Issue published 27 February 2024
Abstract
Bone age assessment (BAA) helps doctors determine how a child’s bones grow and develop in clinical medicine. Traditional BAA methods rely on clinician expertise, leading to time-consuming predictions and inaccurate results. Most deep learning-based BAA methods feed the extracted critical points of images into the network by providing additional annotations. This operation is costly and subjective. To address these problems, we propose a multi-scale attentional densely connected network (MSADCN) in this paper. MSADCN constructs a multi-scale dense connectivity mechanism, which can avoid overfitting, obtain the local features effectively and prevent gradient vanishing even in limited training data. First, MSADCN designs multi-scale structures in the densely connected network to extract fine-grained features at different scales. Then, coordinate attention is embedded to focus on critical features and automatically locate the regions of interest (ROI) without additional annotation. In addition, to improve the model’s generalization, transfer learning is applied to train the proposed MSADCN on the public dataset IMDB-WIKI, and the obtained pre-trained weights are loaded onto the Radiological Society of North America (RSNA) dataset. Finally, label distribution learning (LDL) and expectation regression techniques are introduced into our model to exploit the correlation between hand bone images of different ages, which can obtain stable age estimates. Extensive experiments confirm that our model can converge more efficiently and obtain a mean absolute error (MAE) of 4.64 months, outperforming some state-of-the-art BAA methods.Keywords
Cite This Article
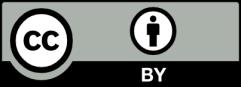