Open Access
ARTICLE
A Novel Eccentric Intrusion Detection Model Based on Recurrent Neural Networks with Leveraging LSTM
1 Department of Computer Applications, Karpagam College of Engineering, Coimbatore, 641032, India
2 Department of Computer Science and Engineering, Karpagam College of Engineering, Coimbatore, 641032, India
3 Department of Data Science and Business Systems, College of Engineering and Technology, SRM Institute of Science and Technology, Kattankulathur, 603203, India
4 Department of Computer Science and Engineering, Sathyabama Institute of Science and Technology, Jeppiaar Nagar, Rajiv Gandhi Salai, Chennai, 600119, India
5 Department of AI Convergence Network, Ajou University, Suwon, 16499, Korea
* Corresponding Author: Jehad Ali. Email:
Computers, Materials & Continua 2024, 78(3), 3089-3127. https://doi.org/10.32604/cmc.2023.043172
Received 24 June 2023; Accepted 15 December 2023; Issue published 26 March 2024
Abstract
The extensive utilization of the Internet in everyday life can be attributed to the substantial accessibility of online services and the growing significance of the data transmitted via the Internet. Regrettably, this development has expanded the potential targets that hackers might exploit. Without adequate safeguards, data transmitted on the internet is significantly more susceptible to unauthorized access, theft, or alteration. The identification of unauthorised access attempts is a critical component of cybersecurity as it aids in the detection and prevention of malicious attacks. This research paper introduces a novel intrusion detection framework that utilizes Recurrent Neural Networks (RNN) integrated with Long Short-Term Memory (LSTM) units. The proposed model can identify various types of cyberattacks, including conventional and distinctive forms. Recurrent networks, a specific kind of feedforward neural networks, possess an intrinsic memory component. Recurrent Neural Networks (RNNs) incorporating Long Short-Term Memory (LSTM) mechanisms have demonstrated greater capabilities in retaining and utilizing data dependencies over extended periods. Metrics such as data types, training duration, accuracy, number of false positives, and number of false negatives are among the parameters employed to assess the effectiveness of these models in identifying both common and unusual cyberattacks. RNNs are utilised in conjunction with LSTM to support human analysts in identifying possible intrusion events, hence enhancing their decision-making capabilities. A potential solution to address the limitations of Shallow learning is the introduction of the Eccentric Intrusion Detection Model. This model utilises Recurrent Neural Networks, specifically exploiting LSTM techniques. The proposed model achieves detection accuracy (99.5%), generalisation (99%), and false-positive rate (0.72%), the parameters findings reveal that it is superior to state-of-the-art techniques.Keywords
Cite This Article
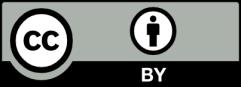