Open Access
ARTICLE
Part-Whole Relational Few-Shot 3D Point Cloud Semantic Segmentation
1 School of Computer Science and Artificial Intelligence, Aliyun School of Big Data and School of Software, Changzhou University, Changzhou, 213164, China
2 Department of Computer Science, University of Aberdeen, King’s College, Aberdeen, AB24 3FX, UK
* Corresponding Author: Yi Liu. Email:
Computers, Materials & Continua 2024, 78(3), 3021-3039. https://doi.org/10.32604/cmc.2023.045853
Received 09 September 2023; Accepted 27 November 2023; Issue published 26 March 2024
Abstract
This paper focuses on the task of few-shot 3D point cloud semantic segmentation. Despite some progress, this task still encounters many issues due to the insufficient samples given, e.g., incomplete object segmentation and inaccurate semantic discrimination. To tackle these issues, we first leverage part-whole relationships into the task of 3D point cloud semantic segmentation to capture semantic integrity, which is empowered by the dynamic capsule routing with the module of 3D Capsule Networks (CapsNets) in the embedding network. Concretely, the dynamic routing amalgamates geometric information of the 3D point cloud data to construct higher-level feature representations, which capture the relationships between object parts and their wholes. Secondly, we designed a multi-prototype enhancement module to enhance the prototype discriminability. Specifically, the single-prototype enhancement mechanism is expanded to the multi-prototype enhancement version for capturing rich semantics. Besides, the shot-correlation within the category is calculated via the interaction of different samples to enhance the intra-category similarity. Ablation studies prove that the involved part-whole relations and proposed multi-prototype enhancement module help to achieve complete object segmentation and improve semantic discrimination. Moreover, under the integration of these two modules, quantitative and qualitative experiments on two public benchmarks, including S3DIS and ScanNet, indicate the superior performance of the proposed framework on the task of 3D point cloud semantic segmentation, compared to some state-of-the-art methods.Keywords
Cite This Article
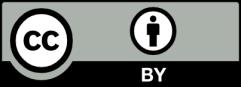