Open Access
ARTICLE
Unsupervised Color Segmentation with Reconstructed Spatial Weighted Gaussian Mixture Model and Random Color Histogram
1 School of Computer and Information Science, Hubei Engineering University, Xiaogan, 432000, China
2 Institute for AI Industrial Technology Research, Hubei Engineering University, Xiaogan, 432000, China
3 Key Lab of Mountain Hazards and Surface Processes, Institute of Mountain Hazards and Environment, Chinese Academy of Sciences, Chengdu, 610041, China
4 University of Chinese Academy of Sciences, Beijing, 100049, China
5 College of Aviation, Zhongyuan University of Technology, Zhengzhou, 451191, China
6 Research Center of Digital Mountain and Remote Sensing Application, Institute of Mountain Hazards and Environment, Chinese Academy of Sciences, Chengdu, 610041, China
7 School of Computer Science, University of Poonch, Azad Jammu Kashmir, 12350, Pakistan
8 School of Computer Science and Information Engineering, Hubei University, Wuhan, 430062, China
* Corresponding Authors: Umer Sadiq Khan. Email: ; Zhen Liu. Email:
(This article belongs to the Special Issue: Advanced Artificial Intelligence and Machine Learning Frameworks for Signal and Image Processing Applications)
Computers, Materials & Continua 2024, 78(3), 3323-3348. https://doi.org/10.32604/cmc.2024.046094
Received 18 September 2023; Accepted 15 December 2023; Issue published 26 March 2024
Abstract
Image classification and unsupervised image segmentation can be achieved using the Gaussian mixture model. Although the Gaussian mixture model enhances the flexibility of image segmentation, it does not reflect spatial information and is sensitive to the segmentation parameter. In this study, we first present an efficient algorithm that incorporates spatial information into the Gaussian mixture model (GMM) without parameter estimation. The proposed model highlights the residual region with considerable information and constructs color saliency. Second, we incorporate the content-based color saliency as spatial information in the Gaussian mixture model. The segmentation is performed by clustering each pixel into an appropriate component according to the expectation maximization and maximum criteria. Finally, the random color histogram assigns a unique color to each cluster and creates an attractive color by default for segmentation. A random color histogram serves as an effective tool for data visualization and is instrumental in the creation of generative art, facilitating both analytical and aesthetic objectives. For experiments, we have used the Berkeley segmentation dataset BSDS-500 and Microsoft Research in Cambridge dataset. In the study, the proposed model showcases notable advancements in unsupervised image segmentation, with probabilistic rand index (PRI) values reaching 0.80, BDE scores as low as 12.25 and 12.02, compactness variations at 0.59 and 0.7, and variation of information (VI) reduced to 2.0 and 1.49 for the BSDS-500 and MSRC datasets, respectively, outperforming current leading-edge methods and yielding more precise segmentations.Keywords
Cite This Article
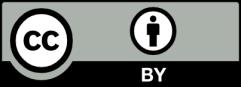