Open Access
REVIEW
Trends in Event Understanding and Caption Generation/Reconstruction in Dense Video: A Review
1 Department of Computer Science, Xiamen University, Xiamen, 361005, China
2 Main Library, Wayamba University of Sri Lanka, Kuliyapitiya, 60200, Sri Lanka
3 School of Information Engineering, Sanming University, Sanming, 365004, China
* Corresponding Author: Abubakar Sulaiman Gezawa. Email:
Computers, Materials & Continua 2024, 78(3), 2941-2965. https://doi.org/10.32604/cmc.2024.046155
Received 20 September 2023; Accepted 06 December 2023; Issue published 26 March 2024
Abstract
Video description generates natural language sentences that describe the subject, verb, and objects of the targeted Video. The video description has been used to help visually impaired people to understand the content. It is also playing an essential role in devolving human-robot interaction. The dense video description is more difficult when compared with simple Video captioning because of the object’s interactions and event overlapping. Deep learning is changing the shape of computer vision (CV) technologies and natural language processing (NLP). There are hundreds of deep learning models, datasets, and evaluations that can improve the gaps in current research. This article filled this gap by evaluating some state-of-the-art approaches, especially focusing on deep learning and machine learning for video caption in a dense environment. In this article, some classic techniques concerning the existing machine learning were reviewed. And provides deep learning models, a detail of benchmark datasets with their respective domains. This paper reviews various evaluation metrics, including Bilingual Evaluation Understudy (BLEU), Metric for Evaluation of Translation with Explicit Ordering (METEOR), Word Mover’s Distance (WMD), and Recall-Oriented Understudy for Gisting Evaluation (ROUGE) with their pros and cons. Finally, this article listed some future directions and proposed work for context enhancement using key scene extraction with object detection in a particular frame. Especially, how to improve the context of video description by analyzing key frames detection through morphological image analysis. Additionally, the paper discusses a novel approach involving sentence reconstruction and context improvement through key frame object detection, which incorporates the fusion of large language models for refining results. The ultimate results arise from enhancing the generated text of the proposed model by improving the predicted text and isolating objects using various keyframes. These keyframes identify dense events occurring in the video sequence.Keywords
Cite This Article
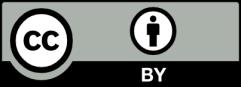