Open Access
ARTICLE
A Sentence Retrieval Generation Network Guided Video Captioning
1 College of Computer Science and Technology, Xi’an University of Science and Technology, Xi’an, 710054, China
2 College of Safety and Engineering, Xi’an University of Science and Technology, Xi’an, 710054, China
* Corresponding Author: Zhenhua Yu. Email:
Computers, Materials & Continua 2023, 75(3), 5675-5696. https://doi.org/10.32604/cmc.2023.037503
Received 06 November 2022; Accepted 27 February 2023; Issue published 29 April 2023
Abstract
Currently, the video captioning models based on an encoder-decoder mainly rely on a single video input source. The contents of video captioning are limited since few studies employed external corpus information to guide the generation of video captioning, which is not conducive to the accurate description and understanding of video content. To address this issue, a novel video captioning method guided by a sentence retrieval generation network (ED-SRG) is proposed in this paper. First, a ResNeXt network model, an efficient convolutional network for online video understanding (ECO) model, and a long short-term memory (LSTM) network model are integrated to construct an encoder-decoder, which is utilized to extract the 2D features, 3D features, and object features of video data respectively. These features are decoded to generate textual sentences that conform to video content for sentence retrieval. Then, a sentence-transformer network model is employed to retrieve different sentences in an external corpus that are semantically similar to the above textual sentences. The candidate sentences are screened out through similarity measurement. Finally, a novel GPT-2 network model is constructed based on GPT-2 network structure. The model introduces a designed random selector to randomly select predicted words with a high probability in the corpus, which is used to guide and generate textual sentences that are more in line with human natural language expressions. The proposed method in this paper is compared with several existing works by experiments. The results show that the indicators BLEU-4, CIDEr, ROUGE_L, and METEOR are improved by 3.1%, 1.3%, 0.3%, and 1.5% on a public dataset MSVD and 1.3%, 0.5%, 0.2%, 1.9% on a public dataset MSR-VTT respectively. It can be seen that the proposed method in this paper can generate video captioning with richer semantics than several state-of-the-art approaches.Keywords
Cite This Article
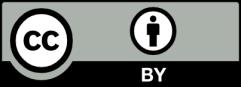