Open Access
ARTICLE
Multi-Task Learning Using Attention-Based Convolutional Encoder-Decoder for Dilated Cardiomyopathy CMR Segmentation and Classification
1 College of Computer Science, Chengdu University of Information Technology, Chengdu, 610000, China.
2 College of Information Science and Technology, Southwest Jiaotong University, Chengdu, 610000, China.
3 West China Hospital, Sichuan University, Chengdu, 610000, China.
4 AI Institute, CuraCloud Corporation, Seattle, 98101, USA.
* Corresponding Author: Xiaojie Li. Email: .
Computers, Materials & Continua 2020, 63(2), 995-1012. https://doi.org/10.32604/cmc.2020.07968
Received 16 July 2019; Accepted 29 August 2019; Issue published 01 May 2020
Abstract
Myocardial segmentation and classification play a major role in the diagnosis of cardiovascular disease. Dilated Cardiomyopathy (DCM) is a kind of common chronic and life-threatening cardiopathy. Early diagnostics significantly increases the chances of correct treatment and survival. However, accurate and rapid diagnosis of DCM is still challenge due to high variability of cardiac structure, low contrast cardiac magnetic resonance (CMR) images, and intrinsic noise in synthetic CMR images caused by motion artifact and cardiac dynamics. Moreover, visual assessment and empirical evaluation are widely used in routine clinical diagnosis, but they are subject to high inter-observer variability and are both subjective and non-reproducible. To solve this problem, we proposed an effective unified multi-task framework for dilated cardiomyopathy CMR segmentation and classification simultaneously, and we firstly update one independent encoder from both recovery decoder and parallel attention path sharing some partial weights. This can encode both task choices into good embedding, but each one can achieve significant improvements respectively from the given embedding. It consists of three branches: extraction path, attention path, and recovery path, which allows the model to learn more higher-level intermediate representations and makes a more accurate prediction. We validated our approach on a DCM dataset, which contains 1155 CMR LGE images. Experimental results show that our multi-task network has achieved accuracy of 97.63%, AUC of 98.32%, demonstrating effectively segmenting the myocardium, quickly and accurately diagnosing the presence or absence of dilation.Keywords
Cite This Article
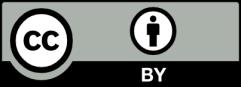