Open Access
ARTICLE
Federated Machine Learning Based Fetal Health Prediction Empowered with Bio-Signal Cardiotocography
1 Department of Computer Sciences, Bahria University Lahore Campus, Lahore, 54000, Pakistan
2 Faculty of Information Technology, Liwa College, Abu Dhabi, 00000, United Arab Emirates
3 Department of Computing, Skyline University College, Sharjah, 1797, United Arab Emirates
4 Department of Computer Science, Taibah University, Medina, 42353, Saudi Arabia
5 Riphah School of Computing and Innovation, Faculty of Computing, Riphah International University, Lahore Campus, Lahore, 54000, Pakistan
6 Department of Engineering and Development, Dubizzle Labs, Lahore, 54000, Pakistan
7 Department of Software, Faculty of Artificial Intelligence and Software, Gachon University, Seongnam-si, 13120, Korea
* Corresponding Author: Khan Muhammad Adnan. Email:
Computers, Materials & Continua 2024, 78(3), 3303-3321. https://doi.org/10.32604/cmc.2024.048035
Received 25 November 2023; Accepted 25 December 2023; Issue published 26 March 2024
Abstract
Cardiotocography measures the fetal heart rate in the fetus during pregnancy to ensure physical health because cardiotocography gives data about fetal heart rate and uterine shrinkages which is very beneficial to detect whether the fetus is normal or suspect or pathologic. Various cardiotocography measures infer wrongly and give wrong predictions because of human error. The traditional way of reading the cardiotocography measures is the time taken and belongs to numerous human errors as well. Fetal condition is very important to measure at numerous stages and give proper medications to the fetus for its well-being. In the current period Machine learning (ML) is a well-known classification strategy used in the biomedical field on various issues because ML is very fast and gives appropriate results that are better than traditional results. ML techniques play a pivotal role in detecting fetal disease in its early stages. This research article uses Federated machine learning (FML) and ML techniques to classify the condition of the fetus. This study proposed a model for the detection of bio-signal cardiotocography that uses FML and ML techniques to train and test the data. So, the proposed model of FML used numerous data preprocessing techniques to overcome data deficiency and achieves 99.06% and 0.94% of prediction accuracy and misprediction rate, respectively, and parallel the proposed model applying K-nearest neighbor (KNN) and achieves 82.93% and 17.07% of prediction accuracy and misprediction accuracy, respectively. So, by comparing both models FML outperformed the KNN technique and achieved the best and most appropriate prediction results as compared with previous studies the proposed study achieves the best and most accurate results.Keywords
Cite This Article
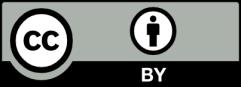