Open Access
ARTICLE
Attention-Enhanced Voice Portrait Model Using Generative Adversarial Network
School of Information Network Security, People’s Public Security University of China, Beijing, 100038, China
* Corresponding Author: Fanliang Bu. Email:
(This article belongs to the Special Issue: Multimodal Learning in Image Processing)
Computers, Materials & Continua 2024, 79(1), 837-855. https://doi.org/10.32604/cmc.2024.048703
Received 15 December 2023; Accepted 28 February 2024; Issue published 25 April 2024
Abstract
Voice portrait technology has explored and established the relationship between speakers’ voices and their facial features, aiming to generate corresponding facial characteristics by providing the voice of an unknown speaker. Due to its powerful advantages in image generation, Generative Adversarial Networks (GANs) have now been widely applied across various fields. The existing Voice2Face methods for voice portraits are primarily based on GANs trained on voice-face paired datasets. However, voice portrait models solely constructed on GANs face limitations in image generation quality and struggle to maintain facial similarity. Additionally, the training process is relatively unstable, thereby affecting the overall generative performance of the model. To overcome the above challenges, we propose a novel deep Generative Adversarial Network model for audio-visual synthesis, named AVP-GAN (Attention-enhanced Voice Portrait Model using Generative Adversarial Network). This model is based on a convolutional attention mechanism and is capable of generating corresponding facial images from the voice of an unknown speaker. Firstly, to address the issue of training instability, we integrate convolutional neural networks with deep GANs. In the network architecture, we apply spectral normalization to constrain the variation of the discriminator, preventing issues such as mode collapse. Secondly, to enhance the model’s ability to extract relevant features between the two modalities, we propose a voice portrait model based on convolutional attention. This model learns the mapping relationship between voice and facial features in a common space from both channel and spatial dimensions independently. Thirdly, to enhance the quality of generated faces, we have incorporated a degradation removal module and utilized pretrained facial GANs as facial priors to repair and enhance the clarity of the generated facial images. Experimental results demonstrate that our AVP-GAN achieved a cosine similarity of 0.511, outperforming the performance of our comparison model, and effectively achieved the generation of high-quality facial images corresponding to a speaker’s voice.Keywords
Cite This Article
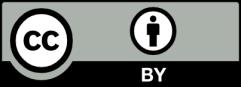