Open Access
ARTICLE
Reinforced CNN Forensic Discriminator to Detect Document Forgery by DCGAN
Department of Electrical Engineering, Soonchunhyang University, Asan, 31538, Korea
* Corresponding Author: Jeongho Cho. Email:
Computers, Materials & Continua 2022, 71(3), 6039-6051. https://doi.org/10.32604/cmc.2022.024862
Received 02 November 2021; Accepted 10 December 2021; Issue published 14 January 2022
Abstract
Recently, the technology of digital image forgery based on a generative adversarial network (GAN) has considerably improved to the extent that it is difficult to distinguish it from the original image with the naked eye by compositing and editing a person's face or a specific part with the original image. Thus, much attention has been paid to digital image forgery as a social issue. Further, document forgery through GANs can completely change the meaning and context in a document, and it is difficult to identify whether the document is forged or not, which is dangerous. Nonetheless, few studies have been conducted on document forgery and new forgery-related attacks have emerged daily. Therefore, in this study, we propose a novel convolutional neural network (CNN) forensic discriminator that can detect forged text or numeric images by GANs using CNNs, which have been widely used in image classification for many years. To strengthen the detection performance of the proposed CNN forensic discriminator, CNN was trained after image preprocessing, including salt and pepper as well as Gaussian noises. Moreover, we performed CNN optimization to make existing CNN more suitable for forged text or numeric image detection, which have mainly focused on the discrimination of forged faces to date. The test evaluation results using Hangul texts and numbers showed that the accuracy of forgery discrimination of the proposed method was significantly improved by 20% in Hangul texts and 5% in numbers compared with that of existing state-of-the-art methods, which proved the proposed model performance superiority and verified that it could be a useful tool in reducing crime potential.Keywords
Cite This Article
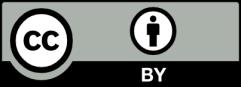