Open Access
ARTICLE
A U-Shaped Network-Based Grid Tagging Model for Chinese Named Entity Recognition
1 Faculty of Information Engineering and Automation, Kunming University of Science and Technology, Kunming, 650504, China
2 Yunnan Key Laboratory of Artificial Intelligence, Kunming University of Science and Technology, Kunming, 650500, China
3 Kunming Enersun Technology Co., Ltd., Kunming, 650217, China
* Corresponding Author: Junjun Guo. Email:
(This article belongs to the Special Issue: Recognition Tasks with Transformers)
Computers, Materials & Continua 2024, 79(3), 4149-4167. https://doi.org/10.32604/cmc.2024.050229
Received 31 January 2024; Accepted 01 April 2024; Issue published 20 June 2024
Abstract
Chinese named entity recognition (CNER) has received widespread attention as an important task of Chinese information extraction. Most previous research has focused on individually studying flat CNER, overlapped CNER, or discontinuous CNER. However, a unified CNER is often needed in real-world scenarios. Recent studies have shown that grid tagging-based methods based on character-pair relationship classification hold great potential for achieving unified NER. Nevertheless, how to enrich Chinese character-pair grid representations and capture deeper dependencies between character pairs to improve entity recognition performance remains an unresolved challenge. In this study, we enhance the character-pair grid representation by incorporating both local and global information. Significantly, we introduce a new approach by considering the character-pair grid representation matrix as a specialized image, converting the classification of character-pair relationships into a pixel-level semantic segmentation task. We devise a U-shaped network to extract multi-scale and deeper semantic information from the grid image, allowing for a more comprehensive understanding of associative features between character pairs. This approach leads to improved accuracy in predicting their relationships, ultimately enhancing entity recognition performance. We conducted experiments on two public CNER datasets in the biomedical domain, namely CMeEE-V2 and Diakg. The results demonstrate the effectiveness of our approach, which achieves F1-score improvements of 7.29 percentage points and 1.64 percentage points compared to the current state-of-the-art (SOTA) models, respectively.Keywords
Cite This Article
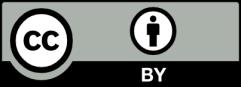