Open Access
ARTICLE
Guided-YNet: Saliency Feature-Guided Interactive Feature Enhancement Lung Tumor Segmentation Network
1 School of Computer Science and Engineering, North Minzu University, Yinchuan, 750021, China
2 School of Medical Information & Engineering, Ningxia Medical University, Yinchuan, 750004, China
3 Key Laboratory of Image and Graphics Intelligent Processing of State Ethnic Affairs Commission, North Minzu University, Yinchuan, 750021, China
* Corresponding Author: Yunfeng Pan. Email:
(This article belongs to the Special Issue: Deep Learning in Medical Imaging-Disease Segmentation and Classification)
Computers, Materials & Continua 2024, 80(3), 4813-4832. https://doi.org/10.32604/cmc.2024.054685
Received 05 June 2024; Accepted 19 August 2024; Issue published 12 September 2024
Abstract
Multimodal lung tumor medical images can provide anatomical and functional information for the same lesion. Such as Positron Emission Computed Tomography (PET), Computed Tomography (CT), and PET-CT. How to utilize the lesion anatomical and functional information effectively and improve the network segmentation performance are key questions. To solve the problem, the Saliency Feature-Guided Interactive Feature Enhancement Lung Tumor Segmentation Network (Guide-YNet) is proposed in this paper. Firstly, a double-encoder single-decoder U-Net is used as the backbone in this model, a single-coder single-decoder U-Net is used to generate the saliency guided feature using PET image and transmit it into the skip connection of the backbone, and the high sensitivity of PET images to tumors is used to guide the network to accurately locate lesions. Secondly, a Cross Scale Feature Enhancement Module (CSFEM) is designed to extract multi-scale fusion features after downsampling. Thirdly, a Cross-Layer Interactive Feature Enhancement Module (CIFEM) is designed in the encoder to enhance the spatial position information and semantic information. Finally, a Cross-Dimension Cross-Layer Feature Enhancement Module (CCFEM) is proposed in the decoder, which effectively extracts multimodal image features through global attention and multi-dimension local attention. The proposed method is verified on the lung multimodal medical image datasets, and the results show that the Mean Intersection over Union (MIoU), Accuracy (Acc), Dice Similarity Coefficient (Dice), Volumetric overlap error (Voe), Relative volume difference (Rvd) of the proposed method on lung lesion segmentation are 87.27%, 93.08%, 97.77%, 95.92%, 89.28%, and 88.68%, respectively. It is of great significance for computer-aided diagnosis.Keywords
Cite This Article
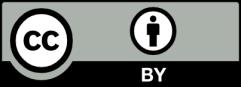
This work is licensed under a Creative Commons Attribution 4.0 International License , which permits unrestricted use, distribution, and reproduction in any medium, provided the original work is properly cited.