Open Access
ARTICLE
Optimal Deep Convolutional Neural Network with Pose Estimation for Human Activity Recognition
1 Department of Computer Science and Engineering, Nandha College of Technology, Erode, 638052, Tamilnadu, India
2 Department of Electronics and Communication Engineering, Kalasalingam Academy of Research and Education, Krishnankoil, 626126, Tamilnadu, India
3 Department of Computer Science and Engineering, St. Joseph's College of Engineering, Chennai, 600119, Tamilnadu, India
4 Department of Electronics and Communication Engineering, University College of Engineering Nagercoil, Nagercoil, 629004, Tamilnadu, India
* Corresponding Author: S. Nandagopal. Email:
Computer Systems Science and Engineering 2023, 44(2), 1719-1733. https://doi.org/10.32604/csse.2023.028003
Received 30 January 2022; Accepted 10 March 2022; Issue published 15 June 2022
Abstract
Human Action Recognition (HAR) and pose estimation from videos have gained significant attention among research communities due to its application in several areas namely intelligent surveillance, human robot interaction, robot vision, etc. Though considerable improvements have been made in recent days, design of an effective and accurate action recognition model is yet a difficult process owing to the existence of different obstacles such as variations in camera angle, occlusion, background, movement speed, and so on. From the literature, it is observed that hard to deal with the temporal dimension in the action recognition process. Convolutional neural network (CNN) models could be used widely to solve this. With this motivation, this study designs a novel key point extraction with deep convolutional neural networks based pose estimation (KPE-DCNN) model for activity recognition. The KPE-DCNN technique initially converts the input video into a sequence of frames followed by a three stage process namely key point extraction, hyperparameter tuning, and pose estimation. In the keypoint extraction process an OpenPose model is designed to compute the accurate keypoints in the human pose. Then, an optimal DCNN model is developed to classify the human activities label based on the extracted key points. For improving the training process of the DCNN technique, RMSProp optimizer is used to optimally adjust the hyperparameters such as learning rate, batch size, and epoch count. The experimental results tested using benchmark dataset like UCF sports dataset showed that KPE-DCNN technique is able to achieve good results compared with benchmark algorithms like CNN, DBN, SVM, STAL, T-CNN and so on.Keywords
Cite This Article
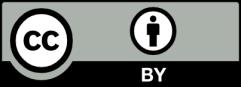