Open Access
ARTICLE
Fire Hawk Optimizer with Deep Learning Enabled Human Activity Recognition
1 Department of Information Systems, College of Computer Engineering and Sciences, Prince Sattam bin Abdulaziz University, Al-Kharj, 16273, Saudi Arabia
2 Department of Information Technology, College of Computers and Information Technology, Taif University, Taif P.O. Box 11099, Taif, 21944, Saudi Arabia
* Corresponding Author: Mrim M. Alnfiai. Email:
Computer Systems Science and Engineering 2023, 45(3), 3135-3150. https://doi.org/10.32604/csse.2023.034124
Received 06 July 2022; Accepted 27 August 2022; Issue published 21 December 2022
Abstract
Human-Computer Interaction (HCI) is a sub-area within computer science focused on the study of the communication between people (users) and computers and the evaluation, implementation, and design of user interfaces for computer systems. HCI has accomplished effective incorporation of the human factors and software engineering of computing systems through the methods and concepts of cognitive science. Usability is an aspect of HCI dedicated to guaranteeing that human–computer communication is, amongst other things, efficient, effective, and sustaining for the user. Simultaneously, Human activity recognition (HAR) aim is to identify actions from a sequence of observations on the activities of subjects and the environmental conditions. The vision-based HAR study is the basis of several applications involving health care, HCI, and video surveillance. This article develops a Fire Hawk Optimizer with Deep Learning Enabled Activity Recognition (FHODL-AR) on HCI driven usability. In the presented FHODL-AR technique, the input images are investigated for the identification of different human activities. For feature extraction, a modified SqueezeNet model is introduced by the inclusion of few bypass connections to the SqueezeNet among Fire modules. Besides, the FHO algorithm is utilized as a hyperparameter optimization algorithm, which in turn boosts the classification performance. To detect and categorize different kinds of activities, probabilistic neural network (PNN) classifier is applied. The experimental validation of the FHODL-AR technique is tested using benchmark datasets, and the outcomes reported the improvements of the FHODL-AR technique over other recent approaches.Keywords
Cite This Article
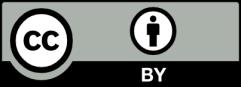